What Are the Infrastructure Implications of AI?What Are the Infrastructure Implications of AI?
We’re far from knowing exactly what network and computing architectures will provide the best performance for AI applications – but we’ve got to figure it out
June 14, 2024
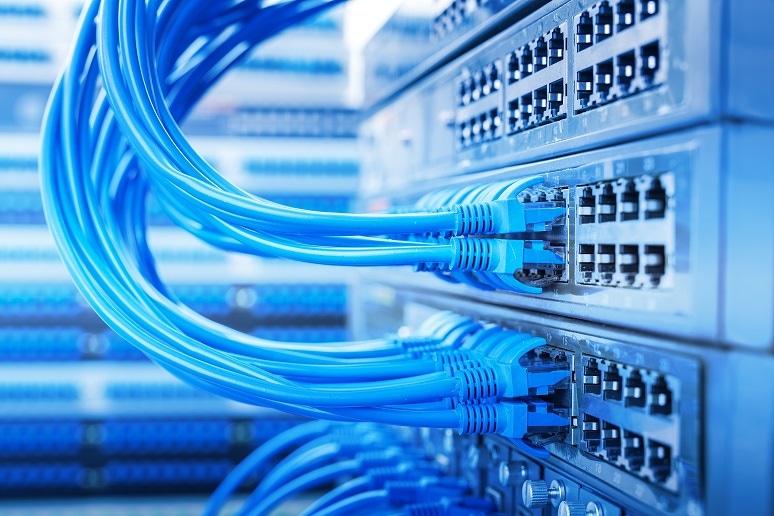
Nvidia’s recent flirtation with the number-two spot in market capitalization reminded me of the moment in 2000 when Cisco spent a short time as the world’s most valuable company. The driver is the same in both cases: Each company manufactures the substrate upon which everyone else plans to build the next big thing. For Cisco, it was the routers and switches that comprise the Internet’s infrastructure, and for Nvidia, it’s the GPUs that are expected to power the AI revolution.
It’s a good reminder that, while use cases, ROI, and governance are critical parts of an enterprise’s AI strategy, infrastructure is an equally indispensable factor. The assumption has been that the largest of the LLMs will run on the hyperscalers’ datacenters, but we live in a hybrid world where enterprises are constantly evaluating which workloads should be centralized and which should run at the edge. AI will be no exception to this reality.
As enterprises learn more about AI technology, they’ll evaluate, among other things, exactly what size language model really fits a particular use case, and they may conclude it makes sense to run smaller LLMs at the edge or even on user devices. They’ll also weigh the perennial factor of security as they evaluate what data is being used to train the LLM, and how that may impact where the LLM should reside.
I’m delighted that we’ll be including a session on the infrastructure and compute implications of AI in our debut Enterprise Connect AI event, taking place Oct. 1 – 2 in Santa Clara, CA. Jim Lundy of Aragon Research will present a session on AI and the Edge: How AI Will Impact Your Network and Compute Architecture, where he’ll address these key questions:
What network and compute strategies should you implement to prepare for the infrastructure, workforce, and business processes of AI architectures?
What types of AI applications/services run best in which environments—cloud vs. edge? And how will your choices about AI applications dictate your requirements around network and compute resources?
What are the best practices in AI at the Edge that will give enterprises a competitive advantage?
Despite all the hype around AI, it’s ultimately just workloads on devices and traffic on networks. It was the same with voice and later video over IP back when service providers and enterprises were building the Internet and intranets on Cisco gear. Nobody really talks about Quality of Service for those applications anymore; the problems that hampered QoS may not have been eliminated, but they were largely brought under control enough to provide acceptable service.
We’re far from knowing exactly what network and computing architectures will provide the best performance for AI applications, but since enterprises are still figuring out which of those applications make sense to deploy, there’s time to figure out the infrastructure. But we will need to figure it out.