Conversations in Collaboration: Slack’s Christina Janzer on How AI-powered Tools May Require Thinking Differently about Productivity, Part 2Conversations in Collaboration: Slack’s Christina Janzer on How AI-powered Tools May Require Thinking Differently about Productivity, Part 2
As we think about AI potentially replacing a lot of the visible busy work, or this “work of work,” we really need to think about productivity in a different way.
March 20, 2024
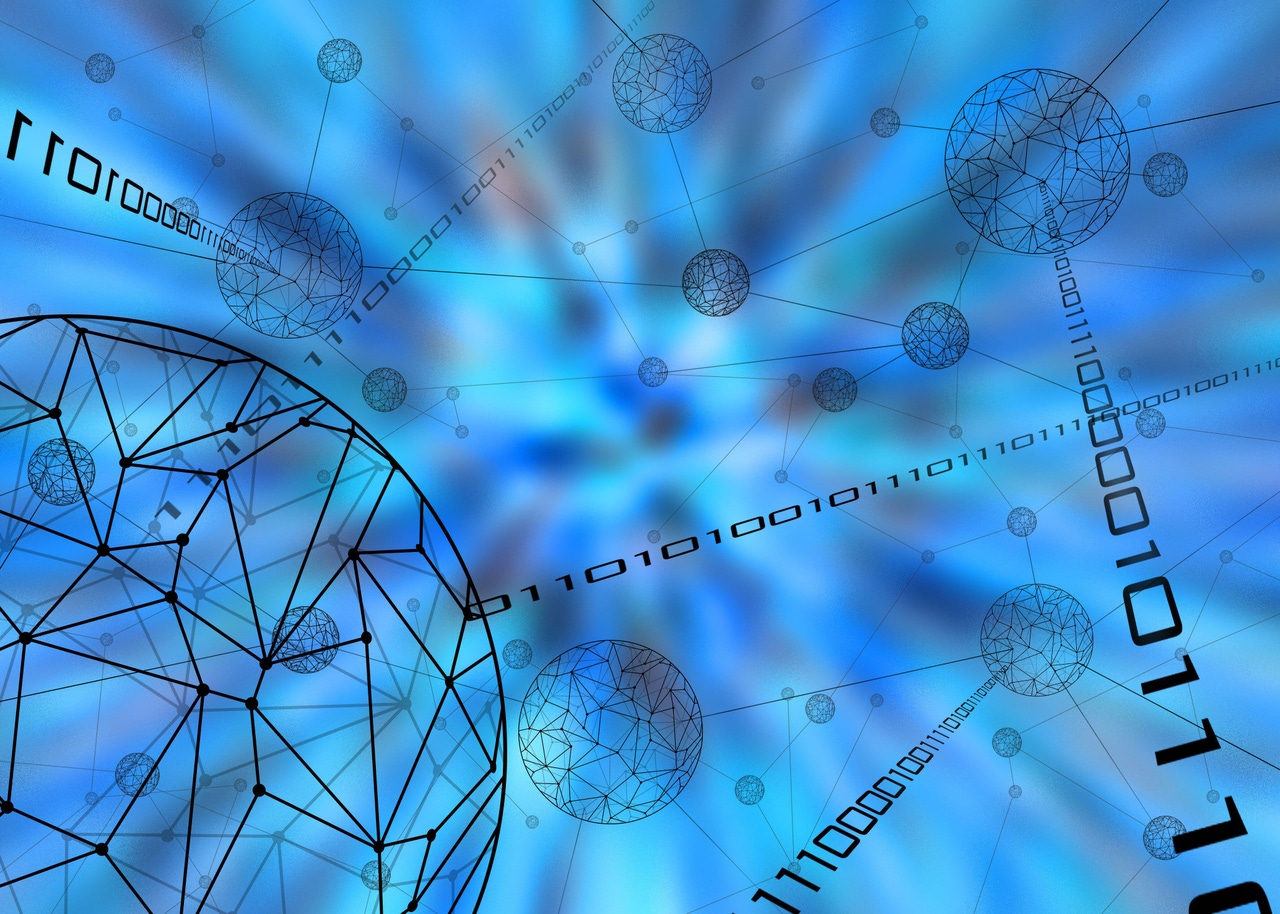
Welcome to No Jitter’s Conversations in Collaboration series. In this current series we’re asking industry leaders to talk about how AI can boost productivity – and how we define what productivity even is – with the goal of helping those charged with evaluating and/or implementing Gen AI to gain a better sense of which technologies will best meet the needs of their organizations and customers.
In this conversation we spoke with Christina Janzer, Slack's SVP of Research & Analytics. In this role, Janzer is responsible for leading global research and product analytics efforts that provide insights about how to make working lives better and more productive. Janzer is also the Head of Slack's Workforce Lab, which studies how to make work better for people, focusing on what drives productivity and great employee experiences; Janzer’s group has just released some research into the use of AI at work – which is the subject of this discussion. (Here is Part 1 of our conversation with Slack.)
No Jitter (NJ): How long have you been looking at the impact of AI? What are some of the major trends you’ve seen in your latest research?
Christina Janzer (CJ): We've been looking at AI specifically since last year, so I can talk a little bit about how we've seen a shift in those trends. What we're seeing, obviously, as you can imagine, is that 2023 was really focused on the promise of AI. In 2024, we're starting to see that take shape.
We surveyed desk workers multiple times last year, and adoption of AI was “stuck” at about 20% of people who were using AI at work. Now we've seen that increase to 25% of knowledge workers using AI at work [from September 2023 to January 2024]. We're also found that 80% feel like AI is helping them be more productive – that’s a high number for how early we are in the journey with AI.
We're also seeing a shift in how people are using AI and the value they're getting. The top tasks are writing assistance, automating workflows, and summarizing content. Summarizing content wasn’t in the top three when we looked at this last year, so that’s a little bit of a shift. We should expect to see more shifts as people start to get more familiar with the technology and as it evolves.
There are also mixed feelings from desk workers: 42% say that they're excited about AI's potential, but the rest are either unsure [31%] or concerned [27%] about what's next.
When we look at the executive perspective versus the desk worker perspective, we see a lot of urgency from executives – 80% reported feeling like they have to figure out how to incorporate AI. It's such a hot topic. Everyone's talking about it, there's this promise that AI is going to improve everyone's productivity. But almost half of desk workers don't have guidelines for how they should be thinking about or how they should be using AI at work. So, there's a disconnect between executive pressure and the lack of guidelines and rules. The enablement isn't there [which helps explain] why there’s concern or a [lack of] overall excitement because there’s so many unknowns.
NJ: Do you have a sense of what AI tools the survey respondents were using?
CJ: For this particular research we surveyed desk workers, they were not specifically Salesforce or Slack customers. We haven't teased apart who from the survey are using which AI tools – some likely use Slack or Salesforce – but we were really trying to understand the broad desk worker trends when it comes to AI so that we can think about that as we develop our own technology.
NJ: How was productivity defined in the survey?
CJ: Since this was a survey, it was a self-assessment of productivity. We asked people how they would define productivity. Their top three answers were:
Quality: How well your work is done.
Effectiveness: Doing the right tasks versus doing busy work.
Project success: The overall impact of your work.
NJ: Did you always ask them to define productivity in the same way over the past few surveys?
CJ: There are a couple of components of this survey. One was trying to tease out what is impacting people's productivity – i.e., is the use of AI impacting your productivity? The way that we asked that question was consistent over time. But as a follow up we also wanted to better understand how people think about productivity and how they define productivity.
Maybe you think about it in terms of the number of hours that you work, whereas I think about it in terms of the output that I produce. We asked that as a separate question, just to understand how desk workers define productivity. But when we're thinking about whether or not AI impacts productivity, we're not getting into sort of the nitty gritty of how to define productivity. That would be a separate thing.
NJ: Are there metrics in place for employees to define or gauge or otherwise quantify how their employees are going to be productive within their corporation?
CJ: We've looked at this in previous surveys. What we've seen in the past is that executives, and this might not surprise you, but executives have continued thinking about productivity in what I would consider a little bit of a backwards way. They really focus on input metrics and activity metrics – so the number of hours that you're working, the number of emails you send, the number of lines of code you produce. I think we need to see a shift away from that.
You could be doing this visible busy work, which maybe doesn’t contribute to the bottom line, but if that's what your manager is going to incentivize, you'll be working on those things. As we think about AI potentially replacing a lot of this visible busy work, this “work of work” … these routine, mundane tasks, we really need to think about productivity in a different way.
So, as you might expect, how individual contributors think about productivity is very highly influenced by how their managers and executives think about productivity.
What we’ve seen in some of our previous research is if you're an executive and you're incentivizing activity metrics and busy work, then people will focus their time on those tasks – even if those tasks aren’t contributing to the bottom line.
I don't think that individual contributors want to think about productivity in the input, busywork type of way. But since we know pretty confidently from our data that the majority of executives still think about productivity in this input way, I think we have to really pay attention to the incentive structure.
NJ: You mentioned that the perceptions of desk worker perceptions toward AI was mixed. Can you provide some insights there?
CJ: Well, first of all, we are early in this AI journey so I think that there's a lot of excitement, but there's also a lack of information – 43% of desk workers don't have guidelines or guidance or rules about how to use AI, so they don't even feel comfortable trying it right. [We found that] people who have guidelines are six times more likely to have experimented with AI.
So without actually having an understanding about what the tool is, how I’m supposed to use it, and how it’s going to impact my job, I can understand why there's a lot of concern there. Until we actually give people more specific guidelines, rules and restrictions, there will be a lot of unknowns and I think that's really what's causing the concern.
I'm recommending to executives that they create a space where people have the guidelines and the structure to be able to experiment with AI and start to have a conversation around it – like how they can use AI for their specific job. There won’t be a “one size fits all.” It will depend on your job, the responsibilities you have or the tasks that you have to accomplish. The sooner that we can get to a place where people feel comfortable trying it out, seeing how it works, seeing what it's good at – or what it's not good at – then people will start to get more excited because they'll have a better understanding about how AI can benefit them.
As an example, one of my researchers did a project that she called “Ashley versus the Machine.” She wanted to understand how she could use AI to make her job as a researcher better. So, she took her research project, broke it up into 10 different steps – how do we figure out what are the right questions that we want to ask, what are the objectives, writing the survey, analyzing the results – the whole process. For each step she did it as a human and then she asked AI to do the same thing.
She was able to get really detailed about the specific steps that AI is actually good at, so in the future she can turn those over to the AI. She also figured out the areas where she needs to remain really involved in what the AI is doing. That task taught her so much and it also gave her so much confidence about how she specifically should be thinking about AI for her job moving forward.
NJ: The models have gotten better just in the past 18 months or so and you mentioned shifting use cases, like with summarization becoming a bigger deal. How much of that is tied to the model getting better versus the person getting more comfortable asking questions of the model? Any ideas there on what could be going on?
CJ: I don't have great data to answer that, but I think what you're identifying is about the tool actually getting better and stronger and then our own understanding and adoption of [how to use the tool] changing.
When we start to use it and we get a better picture of how we could use it or potentially other use cases, then we start using it for different things – or as we experiment with the tool, and we realize that it's not good at that one thing, but it's good at this other thing so let's go that direction.
So while I don't have great data to support this, I do think we need to closely track the strength of the tool and the accuracy of the tool, but also the ways we're actually using the tool to fit the different use cases that are emerging over time.
I also think we need to pay attention to when people have a poor experience with AI and maybe help ensure that they don't give up because maybe the tool just isn't quite ready yet, but it will be in six months.
That's why this constant culture of experimentation is important. If we assume that the way things are today is the way that things will be in three months, then we're going to be left behind.