Reliance on AI Tools Continues to Carry Significant RisksReliance on AI Tools Continues to Carry Significant Risks
It’s important to know that AI outputs are best used when they are used as contributing factors and not relied upon solely for decision making
July 31, 2024
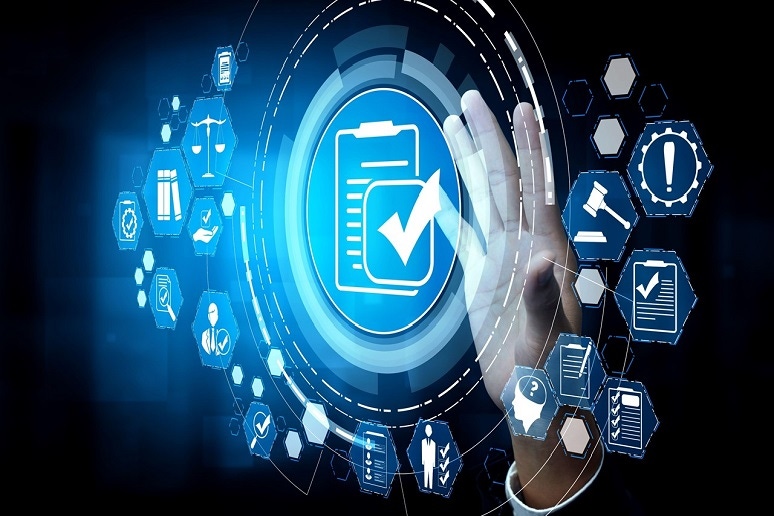
“An Algorithm Told Police She Was Safe. Then Her Husband Killed Her.” appeared in the New York Times on July 18th and it describes the downsides of using AI for life-or-death situations. The article describes an effort by Spanish authorities to rely uponan AI algorithm to categorize and assess the likelihood that previous victims of domestic violence would be assaulted again at the hands of their spouses.
Spanish law enforcement has, according to the Times article, become dependent on a tool using an algorithm called VioGén in an effort to reduce gender-driven violence, “with the software so woven into law enforcement that it is hard to know where its recommendations end and human decision-making begins.”
The article continues, “The tool was created to be an unbiased tool to aid police with limited resources identify and protect women most at risk of being assaulted again.” The word “unbiased” caught my attention immediately. First, people who say or think that they are unbiased do not understand what bias is. We all (and by this I mean people, governments, enterprises and everyone else imaginable) have biases. The challenge is identifying those biases and managing them. Secondly, it’s important to dig a bit deeper to find where those existing biases live within the algorithm. And third, it’s critical to know which values are measured and once determined, how the algorithm weighs them, thus affecting the outcome in the post profound ways possible.
At its best, VioGénhelped police protect vulnerable women and, overall, has reduced the number of repeat attacks in domestic violence cases. But the reliance on VioGén has also resulted in victims, whose risk levels are miscalculated, getting attacked again — “sometimes leading to fatal consequences.”
Spanish authorities are not alone in resorting to these tools in an effort to minimize harm and assist in the determination of sentencing, scheduling of police patrols and, among other things, identifying children who are considered to be at risk for abuse. However, use of such tools is not without significant riskand bias causing undue harm and misidentification.
The system isn’t a complete failure, but at least one woman the system judged as low risk of being killed by a former partner did die because the police chose to weigh the algorithm’s conclusions over the lived experiences of the person who knew her abuser. So the AI-powered system also has not been a ringing success.
Reliance on AI-powered systems raises serious questions about dependence on AI outcomes to determine levels of risk and danger to human life. Enterprise users—proceed with caution.
As we are all acutely aware, humans are capable of making all sorts of serious errors on their own without AI intervention. These errors are made in literally in every aspect of our lives, be they personal or professional. We all make mistakes—sometimes based on solid facts, and other times not so much. But when such errors, with deadly consequences, are made due to reliance on AI-driven outputs, the horrors of these mistakes may be magnified to unprecedented costly (and likely valid) claims of negligence.. How quickly can you say big fat lawsuit?
The Times’high-profilehorror story camefrom Spanish law enforcement, but stateside, governmental authorities have been using a tool called COMPAS (Correctional Offender Management Profiling for Alternative Sanctions). There are multiple tools like this that are used to assess a criminal defendant’s likelihood of returning to criminal activity—or becoming a recidivist post release. There are a number of these risk assessment algorithms in use, some of which have been created by private companies, some by academics, and some by law enforcement agencies themselves. Many states have built their own assessments, and several academics have written tools.
But the problem again is bias. In an often-cited 2016 article published by Pro-Publicathat reviewed one company’s privately developed product, system outputs were often misleading to the point of just plain wrong. Based on a sample of 10,000 defendants in Florida, Black defendants were deemed more likely to be at based at a higher level of recidivism by the vendor’s scoring system than they actually were, while white defendants were often deemed at a lower risk, which is equally untrue.
AI systems may be incredibly useful in processing, sorting, crunching and managing data, but when it comes to interpretation of that data, the danger of mischaracterization and thus inaccurate conclusions drawn from the data is very grave.
To refer one more time to the New York Times article: currently in Spain there are “92,000 active cases of gender violence victims who were evaluated by VioGén, with most of them — 83 percent — being classified as facing little risk of being hurt by their abuser again.” However, since 2007, at least 247 women have also been killed by their current or former partner after VioGén deemed them as facing little risk of future harm, according to figures released by the Spanish government. More than half of the convicted killers were assessed by the system as of low or negligible risk as repeat abusers.
Certainly not all AI tools cause—or result in--harmful outcomes. But it’s important to know that AI outputs are best used when they are used as contributing factors and not relied upon solely for decision making those without the involvement of those people who have both common sense and experience well beyond the numbers themselves. In the Spanish system, reference is made that the AI tool and human decision makers were so intertwined that it was very difficult to determine which was which.
The takeaway is this. AI outputs can be very useful and beneficial. But AI has no common sense or judgment, and in and of itself, without human consideration and analysis, the output is only as good as, not only the original data itself, but the weights and qualities of the factors included in the algorithm itself. And even then, like human analysis, it’s not perfect.
About the Author
You May Also Like