Promise and Perils of Sentiment AnalysisPromise and Perils of Sentiment Analysis
The AI-powered software applications known as sentiment analysis tools can give businesses a leg up in lead generation, customer health processes, and sales forecasting.
June 26, 2024
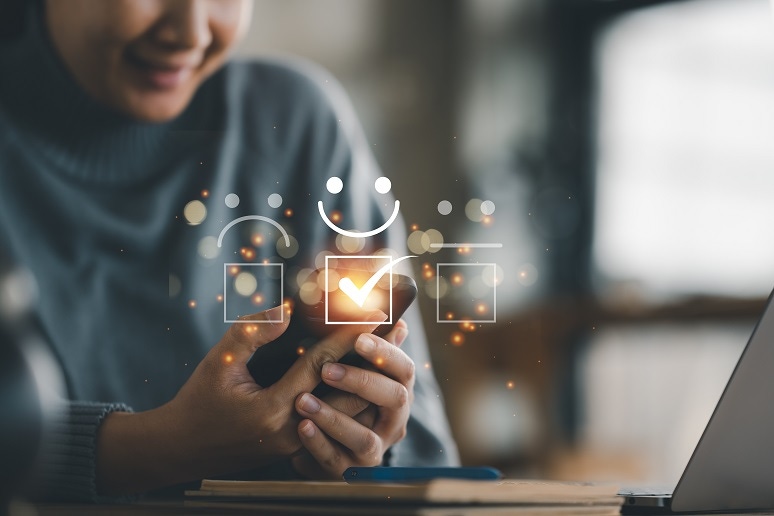
The AI-powered software applications known as sentiment analysis tools, designed to analyze and interpret the emotions, opinions, and attitudes of consumers, can give businesses a leg up in lead generation, customer health processes, and sales forecasting.
These tools use natural language processing (NLP) techniques to understand and categorize the sentiment conveyed in text or voice as positive, negative, or neutral.
Advancements in NLP and machine learning (ML) technologies are enabling a better understanding of linguistic nuances and context cues.
"Sentiment analysis tools empower brands to maximize their customer data as they analyze and deliver insights on customer feedback in real time, allowing brands to react to customer needs quickly," explains Crystal Miceli, vice President of product and industry marketing at Talkdesk.
She explains collecting both positive and negative feedback guides brands to shift their strategies to best meet customer needs.
For example, if a brand receives positive feedback on the checkout process at one store they can implement the same process at other locations, thus improving the customer experience across the business.
By digging into customer sentiment analysis data, brands can also identify common complaints and make changes to improve future interactions.
"Sentiment analysis helps brands deliver a more personalized experience and move the CX from a reactive to a proactive state," she says.
Clearing Language Barriers, Ensuring Transparency
However, misinterpretations of sentiment can lead to wrongly prioritized improvements or innovations, inappropriate responses to customer complaints or feedback, misjudged marketing campaigns, and internal miscommunications.
"Challenges still exist in overcoming language barriers, dialect variations, and evolving slang, requiring ongoing refinement of algorithms and training datasets," admits Jerod Johnson, senior technology evangelist at Cdata.
He says it’s important to prioritize ethics and transparency, informing customers about how and why their data is used and shared.
"Constant moderation and human oversight are extremely important when it comes to sensitive data," he explains.
Data privacy and ethics play a crucial role to ensure fairness and maintain trust when using these tools.
Johnson also recommends businesses that rely on algorithms should disclose data usage practices to consumers to build trust, avoid discriminatory biases, and ensure fairness.
Beware of Biases, Inaccuracies
Miceli explains automated sentiment analysis algorithms can lack contextual understanding, meaning that they will not always identify sarcasm or negation/contradictions.
"This lack of understanding leads to inaccuracies that can negatively impact the customer experience, if not properly managed," she cautions.
For example, technology might interpret, "Great, my package has been lost" as a positive sentiment since the word "great" was mentioned.
Cultural bias of these tools is another major threat to retailers--31% of consumers would stop shopping with a brand if they felt like its AI did not represent or include diverse communities, according to a recent Talkdesk survey.
Johnson says common biases and inaccuracies include cultural biases, contextual ambiguities, and linguistic nuances that may lead to misinterpretation.
"A lack of understanding of sarcasm or slang can result in misclassified sentiment," he says.
Tools may also misinterpret text that contains mixed sentiments, say praising a support team, but disparaging the product itself, leading to inaccurate or inconclusive results.
That's why organizations must incorporate human oversight to validate results and ensure that the datasets are diverse enough to capture various cultural and linguistic nuances.
"It’s important for humans to work alongside AI, rather than be replaced by it because the technology requires human guidance," Johnson says.
He adds it’s important to regularly update algorithms to adapt to evolving language trends and context.
NLP, ML Advancements Boost Capabilities
Miceli notes NLP and machine learning technologies continue to evolve and improve the accuracy of sentiment analysis tools--one of the most important advancements is multilingual and cross-lingual language models.
"These models allow brands to apply sentiment and mood analysis tools to multiple languages with one singular model, saving businesses money and eliminating any language biases," she says.
Deep learning techniques have also greatly improved sentiment and mood analysis as these models can capture nuances of language, which is challenging for simpler models.
"Although the technology has continued to advance, there is still room for improvement," Miceli adds. "Many sentiment and mood analysis tools still struggle to understand specialized language such as jargon."
Zdatny points to new techniques in conversational analytics allowing sentiment analytics platforms to go beyond simply understanding discussed topics.
"Every interaction – be it a chat, post, review, call, or comment – can be automatically analyzed for topic, sentiment, emotion, effort, reason, and intent," she explains.
To do this well, organizations must supplement pre-built topic models with powerful AI that is trained on human conversations from billions of real client and employee interactions.
"Not many businesses have the data to do this well or to specialize in industry-specific challenges," Zdatny says.
Best Practices for Sentiment Analysis Integration
Isabelle Zdatny, head of thought leadership for Qualtrics XM Institute, says like all customer experience activities, implementing sentiment analysis technology requires coordination and collaboration across several different groups.
While customer experience (CX) teams are often the champions of the initiative, they will likely need to partner with their colleagues in IT to implement and integrate the tools with the company’s existing tech stack.
"To derive the most value from sentiment analysis, the insights it produces should be combined with data from other systems around the organization," she says.
This includes operational data (sales, finance, and HR data) and experience data from sources including surveys, market research, and digital channels.
Ideally, these insights should also automatically integrate with the business applications – like Slack, Jira, or Teams – use by different teams, so they can incorporate a better understanding of customers into their normal workflows.
"Along with IT, integrating all these systems together will also require the cooperation of relevant data and workflow owners," Zdatny says.
She adds these types of cross-functional efforts require executive-level engagement and buy-in.
"Leaders should not only allocate resources and drive accountability across these different departments, but they should also set the vision and objective for why acting on these sentiment-rich insights is important to the business," she says.