Power of Babel: The Evolution of Real-Time Translation FeaturesPower of Babel: The Evolution of Real-Time Translation Features
Artificial intelligence has cut down the noise and boosted accuracy to help real time translation flourish – but there’s still more progress to be made.
November 11, 2024
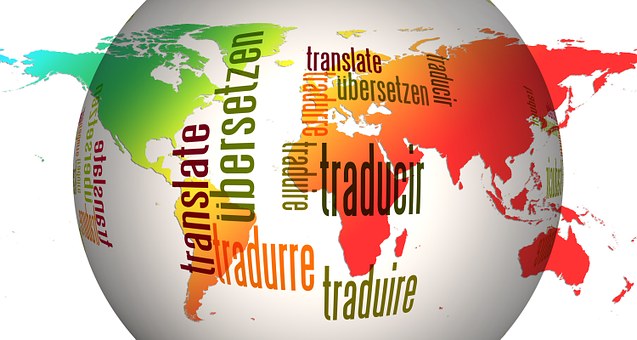
The evolution of real-time translation features has significantly advanced communication by enabling instant accurate translations across languages through sophisticated AI algorithms and natural language processing.
These developments have not only bridged language barriers but also transformed global interactions in business travel and social networking making cross-cultural communication more seamless than ever before.
According to Metrigy, more than 50% of participants look to third-party services to translate meetings into other languages, incurring an average cost of $172 per meeting, per language.
Integrating translation capabilities reduces these costs, increases productivity, and ensures all employees have an equal voice.
This personalized language experience is crucial for fostering inclusive and efficient communication in a globalized workforce.
Automatic speech recognition (ASR) and machine translation (MT) have been one of the earliest technologies in the field of artificial intelligence (AI).
As research and technology have progressed over the decades, the complexity of the tasks for which transcription and translation became available also increased.
Improving Global Communication
Today, real-time speech translation improves communication and breaks down the language barrier, thus enabling participants in virtual meetings to communicate with each other regardless of what language they speak.
Microsoft Teams has integrated real-time translation into its live captions feature, allowing users to select their preferred language from a growing list of supported languages, and other platforms including Google Meet have also begun incorporating translation features into their offerings.
Zoom for example currently offers real-time speech translation for Zoom Meetings, Zoom Events, as well as text translation for Zoom Team Chat—the company’s AI-driven tools can support up to 12 languages.
“For real-time speech translations to be effective, the systems need to operate with low latency, meaning the technology needs to be able to translate as soon as possible to maintain conversational context,” said Sebastian Stüker, director of research science at Zoom.
He explained today’s real-time speech translation systems are a pipeline of several systems, such as voice activity detections, ASR, text normalization, and MT.
“Currently, all systems involved in this pipeline are end-to-end systems based on various kinds of artificial neural networks,” he said.
Stüker noted the switch to these kinds of neural systems has led to a significant improvement in performance at all stages of the pipeline over the previous generation of technology.
Snorre Kjesbu, senior vice president and general manager of collaboration devices at Cisco, said real-time translation features have been incredibly transformative, especially in organizations with a distributed workforce.
“These features help overcome language barriers, enabling companies to focus on finding the best talent regardless of location or native language, thus offering both talent and cost benefits,” he said.
Cisco leverages AI for tasks like removing background noise and ensuring high video quality with low bandwidth to provide accurate real-time transcriptions and translations.
He explained improvements in language models, the introduction of real-time media models, and latency reductions have been pivotal to improve this technology.
Kjesbu said the emergence of multilingual support in large language models and advancements in algorithms, such as transformer models and attention mechanisms, have also increased real-time translation accuracy.
“The main challenge in achieving real-time translations across different languages is ensuring the quality of data being input into the program,” he explained.
This includes the quality of the input signal and high-quality training data including differing accents and dialects.
Another challenge is the inclusion of customer-specific vocabulary, such as technical terms, brand names, and unique jargon.
For example, industry terms like "Jira" or "IPv6" and specific names can be misinterpreted by generative AI models, leading to inaccuracies.
The context in which words are used can vary significantly between languages, making it difficult for translation algorithms to maintain the intended meaning.
“Cultural nuances and idiomatic expressions add another layer of complexity, as direct translations often fail to convey the same sentiment or meaning,” Kiesbu said. “It’s important that as natural language processing progresses, these challenges are addressed to improve the reliability of real-time translations.”
He noted real-time translation tools face significant challenges in handling nuances, idioms, and cultural references across various languages.
A living example of this is Webex’s partnership with Voiceitt, an AI-powered program for people with non-standard speech.
The integration gives people with speech impairments a way to speak and be understood during virtual meetings through innovative AI captioning and transcription.
Limited Language Training Data a Challenge
Stüker pointed out that there are estimated to be around 7,000 languages in the world. Sufficient training data is limited except for very few languages.
“Often languages have individual properties that require special techniques,” he said. “Often, the words that carry the most information in speech are the ones that occur the least frequently in the training data, making them difficult to recognize and translate for the machine.”
He said he believes speech translation systems must become multimodal translation systems that process all available modalities--the slides presented in meetings, typed chat messages, and emoji reactions, to produce the best translation in real-time.
For example, for meeting transcriptions, Zoom recently added the ability to harness optical character recognition (OCR), to use additional context shared via screen share for more accurate and reliable results, as well as the ability to use in-meeting chats for context.
“Human communication is more than just speech but is a multimodal experience that evolves,” Stüker said. “Truly accurate translation is only possible when considering all contextual information available at the time of translation, as well as all existing pragmatic and world knowledge.
Kjesbu said despite the incredible advancements of the past few years, current real-time translation technologies still face several limitations.
Accent and dialect detection remain a challenge, as do issues with meeting participant crosstalk and overtalk, though advancements in speaker detection are helping.
He said future technologies aim to address these issues, enhancing the accuracy and reliability of real-time translation technologies.”
“Handling low-resource languages and local specificities, such as a native English speaker versus a non-native English speaker with a Norwegian accent--like myself--also needs improvement,” Kjesbu said.
About the Author
You May Also Like