3 Reasons Speech Is Becoming Preferred for Self-Service3 Reasons Speech Is Becoming Preferred for Self-Service
A look at why businesses are adopting natural language for customer self-service at such a rapid pace
December 5, 2018
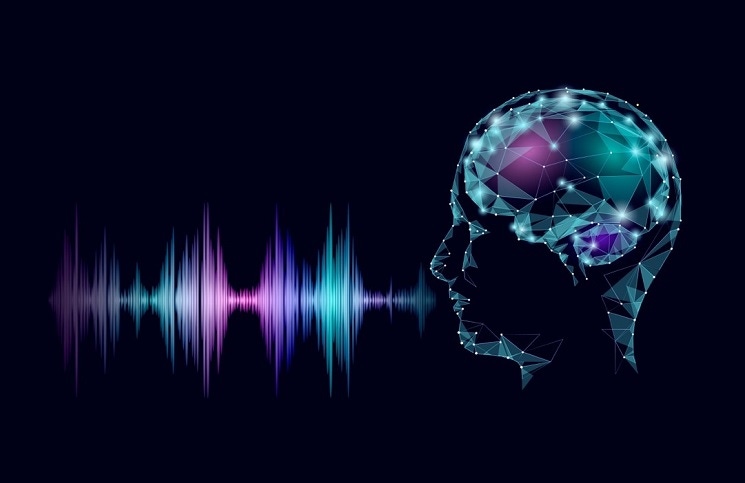
For decades, service managers have understood the benefits of deploying self-service solutions for customer care across multiple service channels. What’s not to like? Self-service solutions give businesses a way to reduce the cost of handling support calls, avoid fines and penalties resulting from lack of compliance, and meet the demands of customers who now expect service anytime and anywhere they want it.
Now, as Donna Fluss, president of DMG Consulting, wrote in a blog post earlier this year, “A remarkable thing is happening in the realm of customer service: After years of rejecting self-service, customers are changing their tune. Consumers of all ages are showing a preference for self-service solutions over talking to agents or using chat boxes, provided they do their jobs well.”
The realization that using a natural language speech interface can improve the speed, effectiveness, and experience of the self-service interaction isn’t new, either.
However, a new generation of cloud-based speech technologies coupled with a new breed of application development tools is making it easy for service organizations of all sizes to build, package, and deploy self-service apps that harness the power of the latest innovations in speech and natural language processing (NLP).
That’s driving a wave of adoption for virtual agents. Gartner has revealed that 25% of customer service and support operations will integrate virtual customer assistant (VCA) or chatbot technology across engagement channels by 2020, up from less than 2% in 2017.
So why are businesses adopting natural language at such a rapid pace? Let’s explore three key drivers.
Lower Cost & Complexity
It’s certainly been possible to build advance natural language speech interfaces for years. In 2000, for example, speech-enabled IVR applications let American Airlines callers ask to “fly from Austin to Boston, next Wednesday at 8a.m.” and Charles Schwab investors to “buy 100 shares of IBM at the market price.”
The problem was, these applications took a lot of time and a whole lot of money to build.
First a company had to buy and host its own speech recognition and text-to-speech servers. Next it had to hire a team of developers to build the application. Finally, it would train the recognition servers and tune the system until it reached the necessarily level of service. The process could take months and cost close to a million dollars, putting speech out of reach of all but the largest call centers.
Speech and NLP have taken a now-familiar path to the cloud. Like other technologies, NLP has become cheaper and more accessible to a wider variety of businesses. Service teams no longer have to manage software, hardware, and equipment, and can now pay for usage based on monthly demand.
In addition, application development cycles have shrunk because cloud vendors like Google and IBM have trained their recognition servers using massive datasets they’ve acquired as millions of users interact with their cloud-based speech services. This further reduces cost and complexity.
Improvements in Quality
The second reason natural language is seeing rapid adoption is that, well, it’s just a lot better. Not a little bit better but dramatically better.
Cloud-based speech services now support open grammars, which means that literally anything said can be translated into text. Previously, voice user interfaces used closed grammars, which meant developers had to predict what a caller might say and then build a set of domain-specific grammars to match variations of requests. For example, a stock trading application would leverage a closed grammar that included a list of ticker symbols and company names. In most cases today, building closed grammars is no longer necessary because the speech servers have been trained to transcribe literally anything a caller might say and cloud-based NLP systems like Google’s Dialogflow can then determine caller intent.
Closed grammars might still be needed in some cases, such as a phone directory application that might need a list of people’s names for better performance. However, Google Cloud and IBM Watson are providing solutions to address this problem. Google uses phrase hints, which is a list of phrases that act as "hints" to boost the probability that words or phrases will be recognized. IBM Watson uses custom models for the same purpose.
Another example of innovation is Google’s Tacotron, a new AI-generated text-to-speech (TTS) system that is almost indistinguishable from a human voice. It uses two neural networks for TTS. The first translates text into a visual spectrogram and the second, WaveNet, reads the spectrogram and creates the final speech. Listen to these audio samples to see if you can tell which is the TTS engine and which is the recorded human voice. I bet you can’t.
Lastly, the number of languages and the variety of voices to choose from has exploded. Businesses can now choose from hundreds of languages… how about Sinhala or Sri Lankan Tamil dialects? And they’re not limited to a handful of voices (please select male or female) but rather you can select from a huge variety.
Advances in Application Development
Recent advances in speech and natural language have been powerful drivers of adoption. But they don’t tell the full story. In order for a business to make use of these technologies it needs an easy way to develop self-service applications that harness the underlying power of the latest AI and speech advances. They also need a way to have those applications deployed within their carrier’s network. Without these tools, businesses are left with little more than a complicated web of APIs forcing them to rely on a team of developers to get their self-service apps (eventually) to market.
One way to do that is through a development platform for intelligent virtual agents. The platform should enable telecommunications carriers to build, package, and deploy natural language applications -- and provide businesses of all sizes the ability to manage and customize virtual agent functionality for their needs.
Like human agents, these virtual agents should have a wide variety of skills, including speech recognition, NLP, TTS, voice biometrics, transcription, and API integration.
And they should be able to perform a variety of tasks. Using a simple drag-and-drop interface, non-technical users should be able to build or select from a set of pre-built tasks, that may include things like biometric enrollment, order lookup, PCI-complaint payment, or queue callback.
Within applications users should be able to select which speech recognition, natural language, TTS, and biometric services they want to use from a variety of vendors like Google Cloud and IBM Watson. There should be no need to license and manage the services from each vendor. And they should be able to switch anytime they choose.
Conclusion
New advancements have improved the quality of speech recognition and NLP while reducing cost and complexity. Those advancements along with a new breed of application development and deployment tools are removing historical barriers to adoption for businesses of all sizes, enabling them to adopt self-service solutions, made simpler and more effective through the use of natural language interfaces.