Conversations in Collaboration: Glean’s Arvind Jain on Searching Enterprise DataConversations in Collaboration: Glean’s Arvind Jain on Searching Enterprise Data
Glean helps solve the problem facing many knowledge workers – they need information to do their jobs, but that information is usually scattered across many internal systems.
February 8, 2024
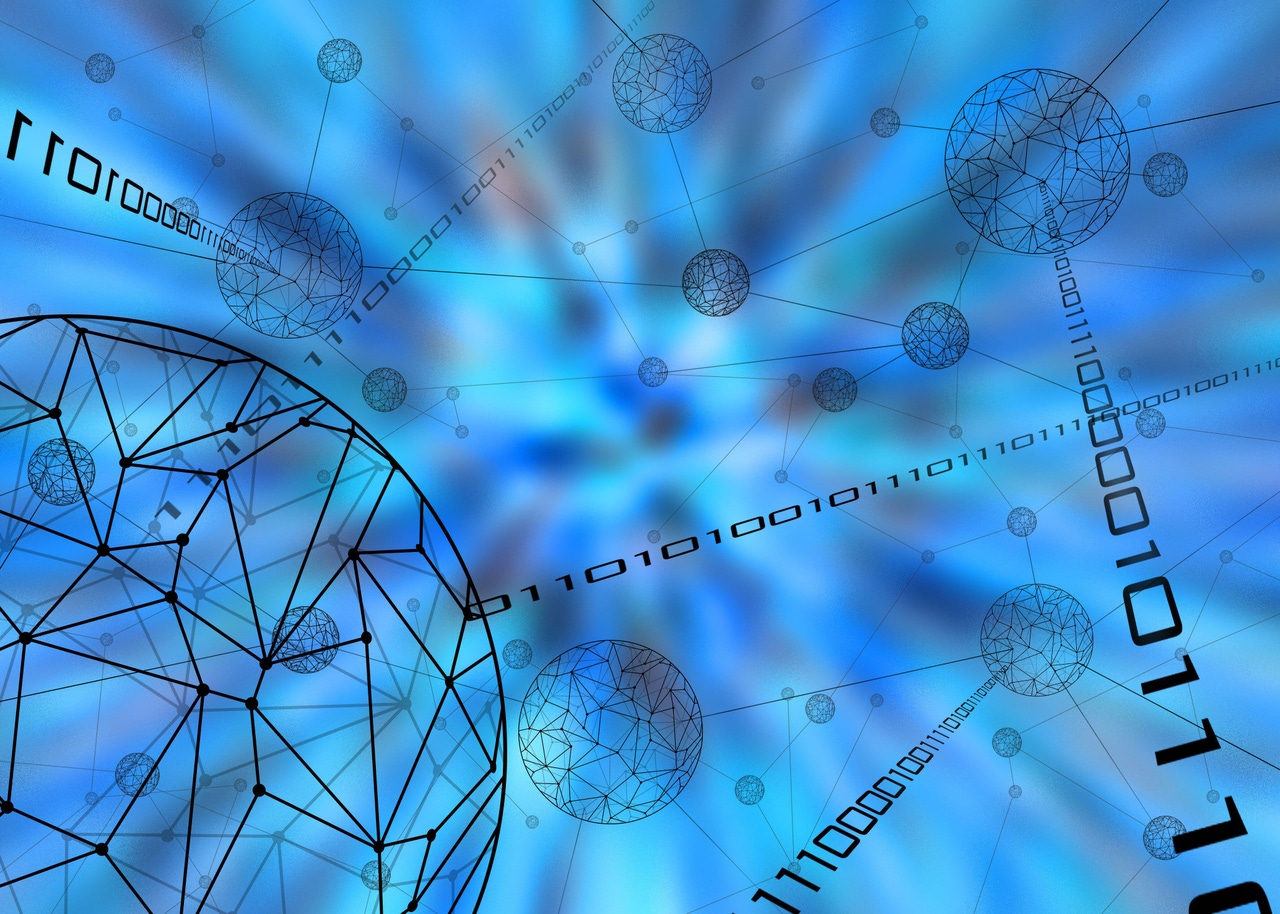
![]() Arvind Jain | Welcome to the debut of the second series of No Jitter’s Conversations in Collaboration. In our initial series, we spoke to executives and thought leaders about generative AI, its impact on collaboration and the contact center, and the need to safeguard the data to which the AI tools were granted access. |
This time, we’re asking industry leaders to talk about how AI can boost productivity – and how we define what productivity even is – with the goal of helping those charged with evaluating and/or implementing Gen AI to have a better sense of which technologies will best meet the needs of their organizations and customers.
This first conversation is with Arvind Jain, the CEO and co-founder of enterprise search and knowledge discovery solution Glean. Prior to Glean, Arvind co-founded Rubrik, a cloud data management company, and served as a Distinguished Engineer at Google, where he spent over a decade leading various teams in Search, Maps, and YouTube. Over the course of this conversation, Jain explained how AI can make enterprise knowledge discovery easier and boost employee productivity by reducing the amount of time workers spend trying to find the information they need to do their jobs.
No Jitter (NJ): Can you start by providing an overview of what Glean does?
Arvind Jain (AJ): Sure. Glean connects all of a company’s knowledge and data and information that’s in many different enterprise systems – Outlook, SharePoint, Teams, Slack, Salesforce. The Glean [interface] gives employees in a company one place to go where they can ask questions [about the information they’re looking for]. So, Glean does what a search engine does on the Internet – surfaces the most relevant documents based on a question and uses generative AI techniques to synthesize a response and answer back to the user. It functions very much like Google Bard or ChatGPT, but inside a company. Basically, it helps employees quickly find information they need to complete their tasks.
NJ: Has Glean always used generative AI or is this a recent introduction?
AJ: We started in early 2019 and, back then, it wasn’t called generative AI – that’s the new term. But, yes, we have always used large language models (LLMs) as part of our core search technology. We don’t do a traditional keyword search to return the most relevant documents.
[The technology behind our search platform is] basically like natural language understanding and processing and [leverages] deep learning-based large language models. It’s a complicated technology but it allows Glean to match user questions with documents even if the way the questions are asked don’t contain the same words as in those documents.
We used to use the LLMs behind the scenes, but the language models have gotten better and better over the last few years. Now you can have the generative model read the documents that contain the answer and then have the model produce an answer for the user.
NJ: You mentioned SharePoint and there’s OneDrive and similar cloud repositories of personal and company data. Is Glean a layer on top of a company's existing platforms?
AJ: Yes, we're a layer on top of all your existing company knowledge and information. Let’s say you’re looking for some information inside these individual systems – SharePoint, Teams, etc. As knowledge workers, we spend one third of our time just looking for information, because there's so much information and it's spread across so many different places inside your company and outside it on the Internet. What Glean does is give you one place where you can search for what you need. You don't have to go into these individual systems.
NJ: Does Glean go outside of a company's datasets or does the user need to switch to a different tool?
AJ: Glean can do that – tap into all the world's knowledge – but that capability depends on what the enterprise wants.
NJ: Microsoft has Copilot, Zoom has AI Companion and WebEx has AI Assistant. ServiceNow has their own assistants. Why would I use Glean rather than one of those tools?
AJ: Every individual tool is adding some AI capabilities inside them, but before we think about AI let’s just think about search. Say you're looking for a recipe to make lasagna. You probably don’t go to recipe.com or some individual websites. You’re more likely to start your journey from Google and you end up in the right place. That's sort of the same concept here.
For example, say I asked for the latest update on a particular customer. In our company, we store some information in Salesforce. Then we had some meetings, and those recordings are stored in a system called Gong. We have discussions about this customer on Slack, then there are engineering feature requests we're tracking in a system called Jira. And then there are emails I've exchanged with them. So, when I ask for that update, none of these individual systems can give me a full, 360-degree summary of the latest on this customer.
Because company information and knowledge reside in all these different systems, oftentimes what you’re looking for can only be generated if you tap into what is in these multiple systems.
NJ: AI’s only as good as the data it has to work with. Does Glean necessitate changes on the enterprise side with how they structure data or store it? Or is it a bridge to get to that place – that centralized, data lake-type repository?
AJ: That’s right. AI technology is only as good as the data that we want to provide to it. With Glean, an enterprise doesn't have to change anything about how it manages its data or where it’s kept. Doing that – making those changes – is very hard. It’s like changing how your business functions to make AI work.
When a company deploys Glean, they establish connections between Glean and their different enterprise systems – and Glean comes with hundreds of integrations to the most popular enterprise systems.
We then pull data from each of those systems and put that into our universal search index so it becomes searchable. After that, we help you build the AI experience, or you can take our ready-to-use AI experience which looks very much like ChatGPT. Either way, users ask their questions in that single place.
Glean will then use our search index and retrieval system to assemble the best pieces of information given the question asked. Then we'll take that information – which could have come from multiple places – and use a large AI model to generate the right answers or artifacts for the user. So, in a way, Glean becomes that centralized AI data lake without enterprises having to do a whole bunch of work.
NJ: Where does that the Glean index reside?
AJ: We can deploy in a public cloud, but we’re very enterprise- and security-focused. So, typically, large enterprises take our product and deploy it within their own cloud environments.
(Editor’s Note: Here are Glean’s terms of service and some background on Glean’s approach to security.)
NJ: How do you charge for it?
AJ: Our pricing model is generally on a per user per month basis. We try to keep it a simple, fixed price regardless of how many systems you connect. We don’t want to complicate it because, to our way of thinking, AI can fundamentally improve productivity for all of a company’s employees. Everybody needs information, whether they're an engineer, marketer, content writer or salesperson. We're trying to reduce that one third of our working time that is spent just looking for information.
Sidebar: About That “One Third” Comment
Jain was referencing this 2019 Forbes article, “Reality Check: Still Spending More Time Gathering Instead of Analyzing,” which cited a 2018 IDC study that found that “data professionals are losing 50% of their time every week — 30% searching for, governing and preparing data plus 20% duplicating work.”
The Forbes article also cited an IDC whitepaper from 2001, “The High Cost of Not Finding Information.” That whitepaper estimated that the typical knowledge worker spent approximately 2.5 hours per day, or roughly 30% of the workday, searching for information. The whitepaper benchmarked the typical knowledge worker’s salary plus benefits at $80,000 per year, and based on this, the paper concluded that an enterprise with 1,000 employees “wastes $48,000 per week, or nearly $2.5 million per year, due to an inability to locate and retrieve information.”
A 2012 McKinsey report, which was linked to in this Glean blog post, focused on social technologies – e.g., Facebook, etc. That report, in turn, cited an IDC study that estimated the average amount of time “interaction workers” spend on various work-related tasks and concluded that approximately 19% of the interaction worker’s week was spent on “searching and gathering information.” The McKinsey report defines an interaction worker as someone in an occupation requiring “complex interactions with other people, independent judgement, and access to information. It is work that is not standardized and is therefore difficult to automate.”
NJ: Do you have a sense how compute, cloud processing, or information moving over the network, might change when the enterprise moves from whatever they have now, if anything, to implementing Glean?
AJ: An enterprise doesn’t need to change anything in terms of what applications they’re running or how they’re storing data. We store our index in a very efficient manner, so Glean doesn't increase cloud or infrastructure cost in any meaningful way. One of the good things about search technology is that it can work with a very small amount of data because we are only interested in the key concepts from documents or other stored files. Typically, we also don’t increase personnel costs which, frankly, is even better. An enterprise can deploy Glean without devoting any engineers to the project.
The value in terms of why people should invest in AI in general is productivity. It’s the first thing on people's mind because AI can bring efficiency. Our customers in their own surveys ask their employees questions like how much time is Glean saving you? Frequently we hear reports that Glean saves people two or three hours of time in the work week.
NJ: Late last year we spoke with an Omdia analyst in which he talked about Microsoft Copilot and the workplaces it can help solve. But one of the questions he had – and I’d echo here – is what happens with “saved time.” I’m not sure it’s possible at this stage in the rollout of Gen AI solutions to fully address that question, but do you have a sense of how enterprises are trying to measure and benchmark these savings now?
AJ: A part of it is, yes, you get work done faster so it leaves room for you to do more things at work. Another part of it is the positive mindset that might come with knowing I have the information I need to do my work. If you think about each individual [job] function, you will start to see that there are actually very hard returns on having access to that information.
Take, for example, the support engineers or the customer support agents inside a company. They are constantly answering questions that customers bring to them. Some of our customers have deployed Glean to their customer care and support teams, and they've found that, as an example, their case resolution time was reduced by 23% and they can reduce their headcount projection – they would have had to grow their team by 20% – but with Glean they no longer need to.
Once you start looking at specific personas and their day-to-day work and how AI and search can bring those efficiencies back to them you can get both those “soft benefits” and the “hard benefits” – like the hours saved – that you can tie to your core business metrics.
Want to know more? Glean’s AI Report
Blair Hanley Frank, Glean product marketing manager, also participated in the call. Here’s how he responded to NJ’s last question:
I just wanted to push back on the idea of productivity as a soft ROI stat. During our customer advisory day, I spoke with a CIO of a very large tech company. He showed me the business case for Glean that he was pitching to senior leadership. His key argument was how many hours Glean would save the company per week, at even a conservative level of adoption. In the first year he was expecting 600% ROI on their Glean spend based solely on productivity estimates. So that's something where CIOs are comfortable thinking about in terms of hard numbers when we're talking about productivity gains.
Glean also released a report on how CIOs and IT leaders view generative AI and its adoption in their organizations. Glean commissioned ISG to survey 224 senior-level IT leaders (VP and C-suite) based across the United States and Europe. Leaders work at companies with at least 1,000 employees and at least $100 million in annual revenue. The survey was conducted in November 2023.
Some of the report’s key findings include the following:
Generative AI projects consumed an average of 1.5 percent of budgets in 2023, and are expected to rise to 2.7 percent in 2024, and 4.3 percent in 2025. Companies with higher revenue tend to plan on investing more in generative AI.
53 percent of leaders said that generative AI will pull budget away from other IT initiatives.
Glean’s study found that skills and talent gaps, along with employee education, are key areas for enterprises to consider when implementing Gen AI. NJ asked for some more context around this finding. Glean’s Frank provided the following:
Most people (including rank and file employees at companies we meet with) still need practice and guidance on how to best leverage prompting in LLMs to generate effective answers. As IT teams implement this technology at their companies, this will require experimentation and education to get right.
For those companies that opt to build their own generative AI applications, developers will need to learn how to work with the relevant LLM APIs and understand how to connect them to other systems to perform actions and interact with users. This can be a rough process, especially connecting an LLM to company data in a secure, permissions-aware manner that generates valuable results.
Some companies may opt to build their own LLMs, which will require even more specialized talent, including dedicated experts in machine learning model creation.
With respect to measuring the return on investment (ROI) of generative AI, Glean’s report found that employee productivity was cited as the number one metric used to evaluate the ROI of generative AI, with 57 percent of respondents saying that it’s a metric they use to measure effectiveness.
And as the following graphic shows, 28 percent of respondents said they’re generating positive ROI from generative AI initiatives, and 31 percent said that they believe they’re generating positive ROI, but don’t have hard data to support this belief. 17 percent said they are not generating positive ROI yet, but they expect they will in the next year; 6 percent said they don’t expect to generate positive ROI in the next year, and 17 percent say it’s too early to tell.
NJ also asked Glean for additional context around the definition for ROI used in the survey. Frank provided the following:
Positive ROI will mean different things to different companies, which is why we did not provide a strict definition. Companies that Glean has spoken with will often multiply a metric like employee hours saved with an hourly rate to find ROI. For other metrics like customer satisfaction, companies may simply be looking for a set improvement rather than linking that to a specific dollar value, but the principle (that fiscal benefits gained from generative AI should outweigh the cost) remains the same.
Note that some of the objectives, such as gaining a competitive advantage, are difficult to measure objectively from an ROI standpoint. Similarly, some of the ROI metrics being measured can address multiple priorities at once (quality metrics could connect to product improvements, for example).
Based on what we’ve heard from companies that we’ve spoken with, gaps in how the metrics align with priorities often have to do with issues in measurability — companies know what they want to evaluate, but don’t always have a method at their disposal that will enable them to do so.