Questioning the Future of Generative of AIQuestioning the Future of Generative of AI
For Generative AI to really improve, it will need to start thinking in a new way — if only it could think.
April 11, 2024
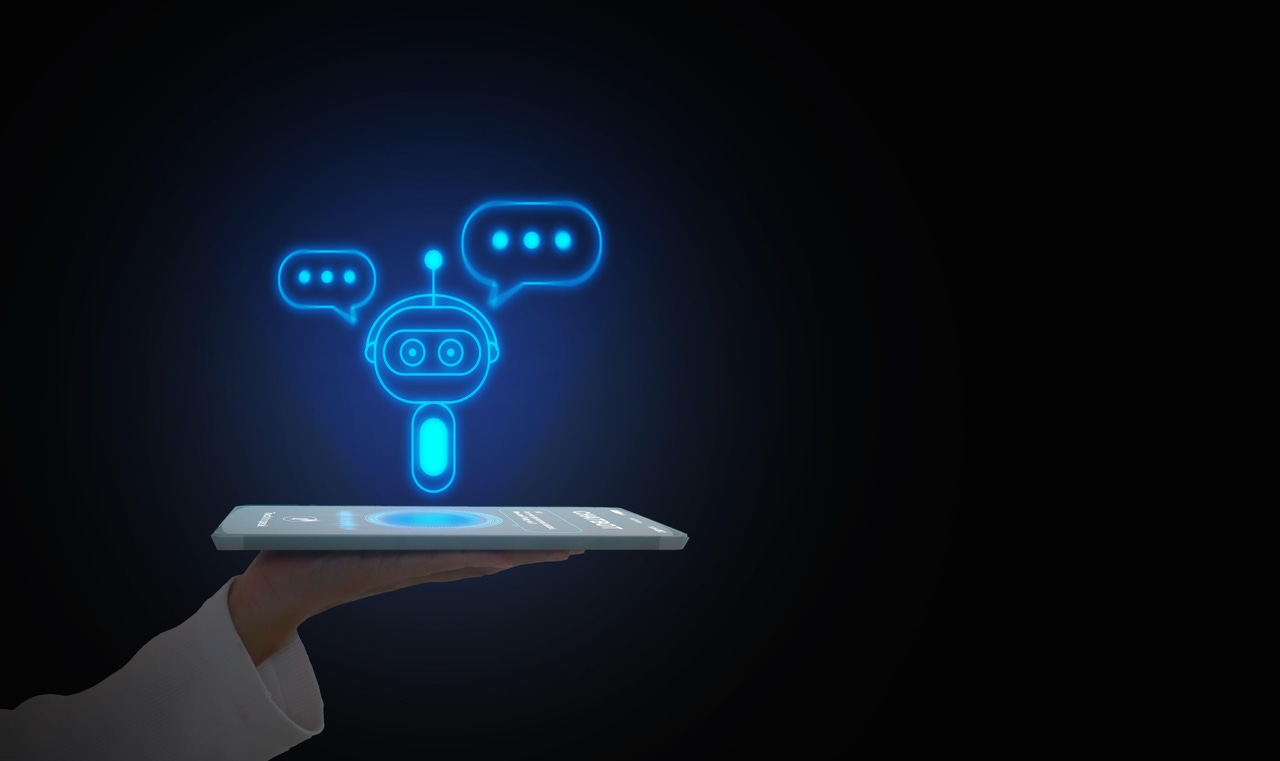
Few developments in artificial intelligence (AI) have captured the collective imagination quite like Generative AI and large language models (LLMs). With their promises of creating art, music, and stories, healthcare diagnoses, and even predicting the future, the excitement is palpable.
Generative and natural language processing (NLP) AI dominated the recent Enterprise Connect conference. There’s tremendous optimism about how AI is already improving enterprise communications, and we are in early innings. The future of AI has never been so optimistic to so many people. There’s a clear consensus that things are going to get much better very soon — or will they?
That may seem blasphemous to some. After all, tech generally gets faster, better, and cheaper over time. But there are some significant differences with generative AI. For starters, we don’t really understand how it works or why it does what it does.
Flipping Numbers Faster May Not Be the Answer
LLMs are a mystery. We don’t understand how they reach any specific answer. If you ask one for a list of the top five of anything, it will provide that list. If you then ask it how it came to that specific answer, it will explain it was ‘trained on a diverse dataset that includes a wide range of information,’ and its response was based on the knowledge and patterns within that dataset. Ask it the same question tomorrow, and the answer might be different.
The architects of these generative AI models that dominated Enterprise Connect really can’t explain how or why these models do what they do. An NYU professor and AI scientist, Sam Bowman, explained it simply: “If we open up ChatGPT or a system like it and look inside, you just see millions of numbers flipping around a few hundred times a second, and we just have no idea what any of it means.” This makes it hard to troubleshoot AI. Researchers are focused on enabling more numbers to flip faster. If we don’t know how it got the something wrong conclusion, it’s not clear how to fix it.
Nor do we understand why these super genius models are so moronic. LLMs have no concept of logic or common sense. They can’t solve many obvious problems. Ask for a picture of an eggplant and it shows sunny-side-eggs-on-stems in a garden. They have problems with simple problems that children get intuitively.
There’s also the hallucination thing. This is the worst kind of ‘not smart’ in that the answers they produce are confidently wrong. It would be so much nicer if they just said, 'error Will Robinson,' but instead they make up reasonable sounding responses and cross their virtual fingers. Who are we mortals to argue? To err is human, as my calculator often reminds me.
The typical response to these mysteries and dilemmas is to wait until the next version. There were, after all, huge improvements by most measures from GPT 3 to GPT 4. Faster, better, and cheaper has so far applied to AI. But it can’t be relied on if we don’t yet understand the tech. Generative AI is a quagmire. Is the answer bigger models, more computing power, more training data, or something entirely different?
Building Without Blueprints
We have accomplished something significant with generative AI. We have machines that can simulate the process of thinking. As a result, many believe computers will soon be thinking. That’s a bit of a leap. We are building without blueprints. We don’t understand why generative AI works, and we don’t understand human intelligence or learning either.
Physicist Emerson Pugh once said “if the human brain were so simple that we could understand it, we would be so simple that we couldn't. Thankfully, the complexity of our brain is so great that we are not simple and neither, therefore, is the task of understanding it.” In developing artificial neural networks, we are piling guess upon guess about the very process of thinking.
There is no evidence or logic or anything beyond hope that the next generation of these ‘simulations of thinking’ will be significantly better than what we have today. More training seems suspicious, we have already fed them most of the Internet, and these models still, inexplicably, can’t solve a Sudoku puzzle.
Ingesting more of the Internet doesn’t appear to be the missing link, especially since it is full of questionable content. Generative AI will soon be the biggest source of dubious content that it learns from. Consider that generative AI caused Amazon to cap the number of self-published ‘books’ an author can submit to three books per day. It's inevitable that the output of today’s LLMs will be training fodder for the neural networks of tomorrow.
There’s now a term for this. Jathan Sadowski coined "Habsburg AI" to describe models trained on the output of another model. Just like repeated photocopies, the results are not what anyone wanted. For Generative AI to really improve, it will need to start thinking in a new way — if only it could think. We are on a curve for faster and cheaper, but something has to change to realize better.
Generative AI has already been transformative. Perhaps we should be satisfied with summaries (meetings, calls, texts, topics, and more) that are 85ish percent accurate. Generative AI has democratized Cliffs-Notes-like summaries better than anything before it. In summary, there’s plenty to celebrate regardless of future improvement.
I hope this post gave you something to think about — whatever that means.
Dave Michels is a contributing editor and analyst at TalkingPointz.