Improve CX by Breaking Down Data Silos and Functional BarriersImprove CX by Breaking Down Data Silos and Functional Barriers
A NICE webinar on CX and AI discusses how and why organizations should not only break down data silos but functional divides among internal teams.
August 22, 2024
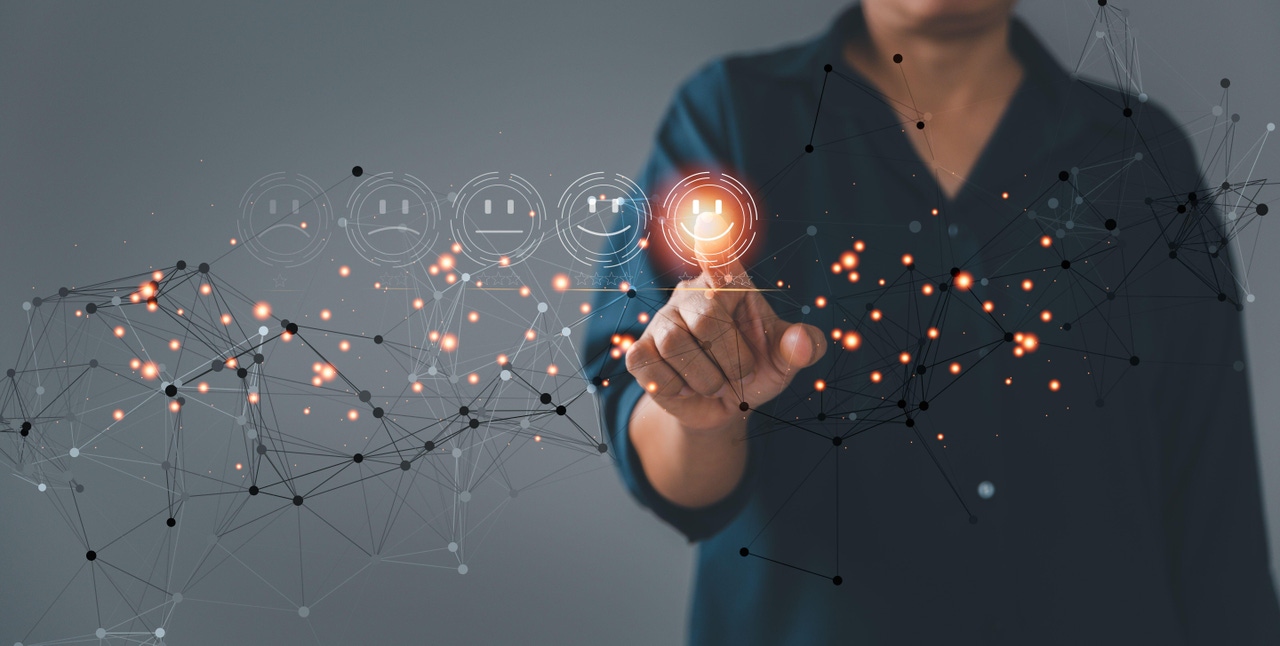
On August 20, 2024, NICE kicked off a three-part webinar series called CX AI Realized. The first webinar, How to Achieve CX Excellence: Cutting Through the AI Hype to Deliver CX Outcomes (recording available now), featured Bradley Shimmin, Chief Analyst, AI & Platforms at Omdia, and Michele Carlson, Director, Product Marketing at NICE.
According to Carlson, customer experience (CX) is no longer contained within the contact because the data it generates (and uses) can be leveraged across the organization – from the laggards to the innovators, as illustrated below. Carlson emphasized that this is a progression, where organizations move from a ‘data silo’ approach to one in which all the different types of data can be integrated and leveraged to improve CX overall – as well as improving service and support, driving revenue, and understanding customers better.
“The goal is not to use AI. The goal is to do what you're doing – and do it more efficiently, better and improve the customers’ experiences. AI is a way to do that,” Carlson said. “The first step in that direction is to think about what data you have because your AI is only as good as the data that is available to it.”
According to Shimmin, “Companies should look at creating what we call a data fabric breaks down all the data silos across the different departments and across the different AI use cases.” Some of those use cases are illustrated in the following slide which presents results from a recent Omdia survey. The purple bar denotes ‘widespread adoption’ of Gen AI in that category whereas the smaller gray bar denotes ‘limited’ uptake. The top three are: IT (including security), cybersecurity and customer support.
Shimmin noted that the purple outpaces the gray in the top two categories, because Gen AI can take a lot of unstructured data and pull knowledge and insight from it for things like anomaly detection in log files (hence the security deployments). Contact centers have long used natural language processing (NLP) and that data can be used the foundation for Gen AI use cases.
The following Omdia slide illustrates how understanding and helping customers solve their problems led the measurable outcomes as ranked by enterprise IT practitioners. “There's still room to grow in terms of taking the entirety of your data – the representation of your company in data form – and utilizing that in a smart manner across a lot of different use cases and a lot of different departments within your company to achieve some form of ROI,” Shimmin said.
Shimmin continued, saying that “It's easy for enterprise practitioners to stand up a chat bot and swap out their existing support bot for a better one. But you have to set up the chatbot in the right way, using the right data – and train it on the right use cases so the chatbot knows the right answers.”
Part of that process can involve some combination of understanding the data used to pretrain the model, the data used to fine tune the model so that it understands the given company’s business, or pointing the model, so to speak, at a specific data set for retrieval augmented generation (RAG), implementing guardrails to constrain the AI, as well as implementing responsible AI practices.
Carlson said that while it’s critical to break down data silos, that same thinking should be applied to an organization’s internal teams and functions. The information powering the chatbots and virtual assistants comes from “your knowledge base, customer support, and the sales and marketing teams,” Carlson said. “This is why it's crucial to think about [each of] these pieces as parts of a whole versus separate use cases.”