Conversations in Collaboration: Calabrio’s Joel Martins on How AI Boosts Contact Center Efficiency and EffectivenessConversations in Collaboration: Calabrio’s Joel Martins on How AI Boosts Contact Center Efficiency and Effectiveness
Calabrio’s CTO talks about current AI implementations in interaction benchmarking and scheduling and provides a glimpse of how generative AI can help contact center managers become more efficient in evaluating quality customer interactions.
August 9, 2023
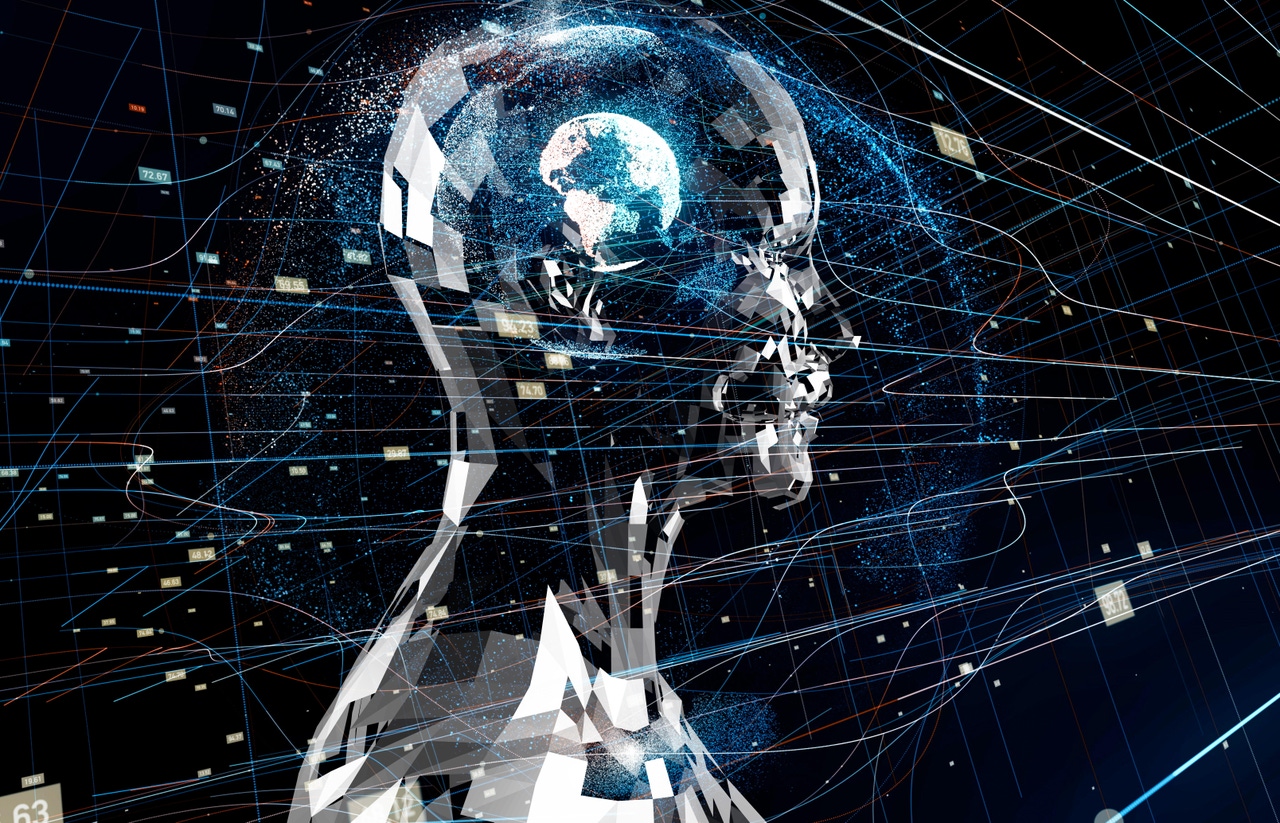
Welcome to the fourth of our Conversations in Collaboration. We began with Cisco's Lorrissa Horton, who talked about the company's approach to data security in AI. No Jitter (NJ) then spoke with Google Cloud’s Behshad Behzadi who spoke about the use of generative AI (Gen AI) at Google, in its Contact Center AI offering and, more broadly, across enterprises. Next, was Amazon Connect’s Pasquale DeMaio who discussed Connect’s history of AI usage and how it’s used in their contact center offering.
(Editor's Note: Artificial intelligence has a lot of specific, descriptive terms. Download our handy guide to AI vocabulary here.)
![]() | For this Conversation, NJ spoke with Joel Martins, Chief Technology Officer, who leads Calabrio’s companywide product, development, and technology teams. Martins joined Calabrio in May 2023 to spearhead Calabrio ONE’s true-cloud AI solutions. Prior to joining Calabrio, Joel served as CTO at Apryse, Social Solutions, and MicroEdge. |
No Jitter (NJ): Maybe we could start out with what Calabrio means by “true cloud?”
Joel Martins: Sure. For us, "true cloud" just means that solution is built as “cloud native,” so we're leveraging the ability to scale horizontally. We offer a multi-tenant service so our customers get the benefit of additional resiliency and scalability because we can automatically scale up and down based on load.
NJ: Can you talk a little bit about where AI in general, and maybe generative AI in particular, fits with your products?
Martins: Sure, so as you know, we're a performance workforce solution provider for customer centric contact centers. Our goal is to help our customers understand their interactions [with their customers], as well as manage and schedule their contact center resources – the agents. AI is already part of our solution today and has been for upwards of a decade.
The way I look at the recent generative AI push is that ChatGPT especially has demonstrated to the world the power of cloud computing. It’s machine learning on steroids leveraging the dataset of essentially all public data information up to a certain point in time. We have an OpenAI beta going and there's more we can do in the AI space, obviously, but I see the recent developments as a continuation rather than something brand new.
NJ: There's a distinction between so-called “predictive AI” and machine learning techniques versus generative AI-based solutions. Is that a legitimate way to describe the difference between the two different types of AI? And what is it that Calabrio’s been doing?
Martins: Yeah, I think [that description is] fair. As for what we’re doing, our customers rely on us to evaluate the interactions they have with their customers. So that they can look at all their customer interactions and say, okay, these went well, these didn't. And then for the ones that didn't go well, look at them and figure out why.
As you can imagine, especially in large contact centers, it is impossible for a human to evaluate every single interaction with a customer and review it. That would take the same amount of time as all those interactions did.
We have automated [customer interaction] evaluation in our products that uses machine learning. What our customers do is create questions that are relevant to their industry or what they want to know about how the interaction went. It can be general things like, "Did the agent ask the person how they're doing today?” or to something more specific [to a particular sector] like " Did the agent use a phrase that indicates that the agent understands that this is a serious matter?"
Each of those questions get scored with our machine learning algorithm. Then we aggregate those scorings, and over time we curate them, and the dataset gets better and better all the time. Basically, we're able to provide accurate “here's how your calls went” autoscoring [which can also be referred to as interaction benchmarking] and that's been in place for many years.
NJ: That autoscoring, or "interaction benchmarking," is based on the kinds of questions the agents are asking?
Martins: Based on what the contact center managers would like the agents to ask or not ask, yes. And our dataset keeps on getting bigger and so we can refine the [interaction benchmarking].
Where the OpenAI beta comes in – and we’re in beta with I think 12 customers – what it does really well is say you're a manager in a contact center, and you look at your automatically scored evaluations and say, “There were four calls here that didn't go very well I wonder what that was about?” Thanks to the OpenAI integration, that manager can click a button and receive a three-sentence summary of what happened on each of those particular interactions.
Or let's say you had a 45-minute interaction between an agent and a customer. If you're a quality manager, you want to know what happened on that call, right? Well, same thing. Click a button and get a three to five sentence summary of what happened – which means that the quality manager, who’s our primary customer, doesn’t have to go read the transcript, or listen to the audio. I mean, they can if they want to, but initially they can just read the summary and then decide what to do. It saves them a lot of time.
And that’s for any interaction where we have the text – so obviously if you just have the audio, you have export that to text first. And we’re already working with customers to integrate their chat interactions, as well.
NJ: With this implementation you’re describing, can the contact center just go in and have every interaction summarized without determining their questions/terms in advance? Then they get to see what falls out of the interactions over time? Is that a possibility?
Martins: Actually, that’s something we’re very likely going to implement soon. Our intention is to use what we call context tagging and sentiment analysis. Essentially, it’s the categorization of the different types of calls and then differences within those calls. So, more generally, what happened and what types of questions were asked.
If you're a manager in a contact center, and you want to figure out what's going on, you're probably going to – based on our research, at least – start your inquiry like all of us, with a search. And you'll search for phrases, for example.
What we're envisioning is somebody could use this search function and we would give that manager all the interactions that use that phrase, but we would have already tagged everything with different categories. So, if they find what they're looking for, then they can easily run a report query for everything with that category and then continue their analysis from there.
NJ: Does that require additional disclosures to end customers or opt ins or anything like that?
Martins: All of our data that goes into an LLM is anonymized. And that's something that [our customers] can opt out of if they don't want their data to go into an anonymized model. That's a contractual item for us. If a prospect or customer doesn't want their data to be used at all, then that's what they can do.
NJ: So how does that work with the different contact center vendors you have partnerships with – they all their own large language models that they either developed themselves or have their own partnerships?
Martins: That’s not something that's come up with the vendors in my experience – probably because our partnerships are with the CCaaS and other contact center vendors. They are handling the contact center-to-human customer interaction while we provide the overlay doing quality management and workforce management. We use large language models to essentially make your quality management evaluations more efficient. So, our partners’ use cases are different from ours – and there’s exceptions to this statement of course.
NJ: Any thoughts or advice maybe to folks who are looking at or evaluating systems that incorporate AI – on security practices, privacy practices?
Martins: I think first and foremost – and this the same thing I do with all my vendors – is make sure that [the people evaluating AI systems] have a rigorous security posture, that they have procedures in place and that they have third party attestations to verify it. We've got a SOC Type 2 certification, for example – many people do – and we're in progress on a FedRAMP certification now. Some of those third-party attestations are helpful because they always involve an audit.
I think it's extremely important for anybody working with vendors to understand how their data is being used and if it is becoming part of a broader data set or not, and if that's okay or not. That's certainly something we take extremely seriously. As we talked about, [our] baseline is that all data is anonymized and then our customers can opt out if they don't want any of their data going into a language model. And even then, for us, those language models are proprietary to Calabrio.
NJ: Can you talk a little about how AI is used in your workforce scheduling product?
Martins: At its core, our workforce management is machine learning. Our core piece of intellectual property is the ML that does the predictive analysis: Do you have enough agents? The follow-on is the scheduling of agents to work at certain times. Now, also built into that is the ability for agents to request to not work or take time off – maybe a doctor's appointment comes up and they need to take two hours off tomorrow or something. That interaction is all handled by a virtual bot within our product, so the agents at that point are interacting with the AI-powered virtual bot in the product, not a scheduler person. All that complex scheduling is just handled within the software.
To help contact centers predict if they're going to have enough people to handle the load and then help the contact center run the schedules. It may seem pedantic, but it's very complex. And it's only gotten more complex in hybrid and virtual working environments – and the gig environment culture, as well. [Mostly] gone are the days where every contact center is a building and everybody's there in three shifts, 24 hours a day, right? Now it’s a lot of hybrid, remote, some part-time staff, etc.
NJ: Why does a hybrid workforce or having contact center agents working from home make the whole scheduling process more difficult?
Martins: I think it boils down to people – employees – having an expectation for more flexible hours. And as you know, people expect more schedule flexibility today than they did four years ago. Everybody's not on a clean cut, eight-hour shift anymore – which makes scheduling contact center agents much more complicated.
I just read a report that makes a direct link between employee happiness and customer experience. If you're somebody running a contact center, what's top of mind for you? These days it’s keeping your agents satisfied. Because that's going to impact your ability to properly service your customers.
Want to know more?
Check out these articles and resources:
Enhancing Agent Experience, Engagement, Empowerment: With the right tools and information to best serve customers, all contact center agents — including those working from home — can become more engaged and empowered.
Use Advanced Analytics to Get the Full Picture of Your CX Performance: Root your technology decisions in quantifiable, verifiable evidence – invest in a customer insights and analytics program
Managing AI Tools Requires More Data Transparency: When deploying products and services with AI embedded into them, organizations must be sure that they can avoid unintended consequences from biased algorithms, faulty training, or incomplete parameters.
About the Author
You May Also Like