Will Nvidia Accidently Disrupt the Cloud Video Market?Will Nvidia Accidently Disrupt the Cloud Video Market?
Nvidia Maxine is already used in several UCaaS solutions but will it gain universal traction?
May 3, 2021
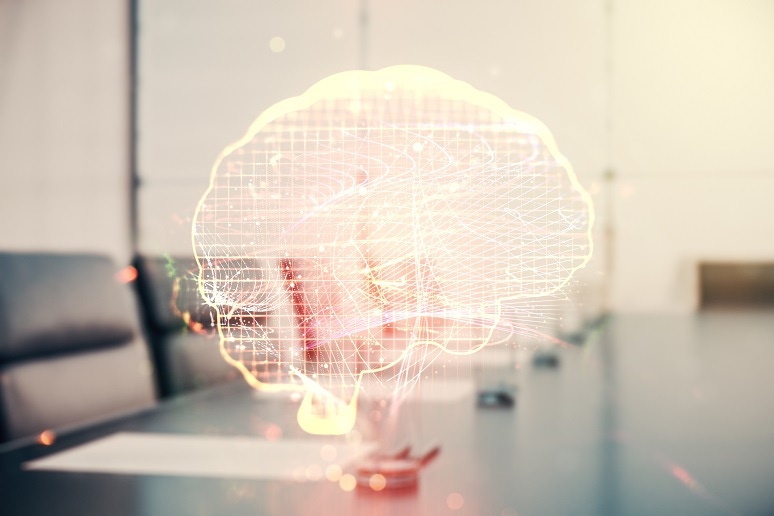
Earlier this month, Pexip and Avaya caught my eye with press releases that both referenced reliance on Nvidia Maxine for critical audio noise removal during video meetings. While other techniques exist for noise cancellation, the current state-of-the-art methods rely on AI to provide this key and potentially differentiating function. Noise reduction is one of the many AI-enabled capabilities Nvidia Maxine offers that will impact how we do virtual meetings now and in the future.
Maxine has been described in No Jitter previously. My point in discussing it again is to illustrate what I think may be the beginning of a tectonic shift in how video endpoints and video services are provided. Not only does Maxine level the competitive landscape between companies that have invested millions of R&D dollars in audio/video computation research and service development and those who haven’t, it also lowers barriers to entry by newcomers that may pull an innovator’s dilemma trick and upstage current incumbents with new video endpoints and services, much like Zoom recently did.
As an example, consider that Cisco closed on the purchase of BabbleLabs in September 2020. BabbleLabs created what I’m going to call an AI-based audio filter that removes unwanted noise from digital audio streams. Cisco recently released the BabbleLabs capabilities in its Webex endpoints, and from the demos at Cisco Live, it is really impressive. At the same time, Cisco was going the acquisition route; Avaya incorporated Nvidia Maxine AI-based audio noise reduction into Avaya Spaces in October 2020.
So, what is the difference between what Cisco and Avaya did? Two subtleties exist: One is where the AI runs, the other is where the AI algorithm comes from. Cisco uses Nvidia GPU chips in its hardware endpoints running its own (BabbleLabs) AI algorithms for noise cancellation. Avaya uses Nvidia GPUs and Nvidia Maxine’s AI noise cancellation in its Spaces cloud service (on the server-side). Nvidia GPUs are involved in both, but Avaya uses Maxine and Cisco doesn’t.
As an aside, GPUs are graphics processor units that massively accelerate the matrix manipulations required for AI-based algorithm development and execution. Nvidia GPUs are widely used for AI processing.
Endpoint-Side or Server-Side: Where Should the AI Run?
This brings up the question as to where should the AI processing be done? To prepare this article, I reached out to both Cisco and Pexip to get more input. These two providers handle media quite differently.
As mentioned, Cisco provides GPUs in its hardware video endpoints, and most of the AI is applied to the media streams in the endpoint. In the Cisco Webex service, the audio and video streams are switched or routed to other endpoints, meaning that in the Webex backend, these media streams are not decoded (unless special processing, like speech-to-text, is invoked). If a Cisco software endpoint is connected to the meeting, like a PC or mobile phone client, the GPU-enabled AI is not available.
Pexip, on the other hand, does not provide endpoints, rather it offers a cloud service that can accept input from nearly any endpoint. The Pexip service decodes the audio and video streams and performs the AI processing on all of the media streams in the backend service regardless of which endpoint is used; it then re-encodes the audio and video streams, creates a composite image, and sends this composite media stream back out to each endpoint.
Pexip stated that running the AI algorithms on the server side has its own advantages:
AI can be applied to any video image regardless of which endpoint originated the image. AI algorithms can even be applied to legacy hardware endpoints and video coming from soft clients and mobile devices. Pexip uses this for its Adaptive Composition capability, where individuals and groups in a meeting are sized appropriately.
Having the GPUs in the cloud, as opposed to having a GPU built into every endpoint, does create some economic benefits. For instance, endpoints are then less expensive.
Endpoints with GPUs built into them will need software updating as AI algorithms change and improve. However, for the cloud-delivered AI, an update like this isn't required.
On the other hand, Cisco pointed out it doesn’t make sense to run some features on the server side. Some examples include:
In situations where latency is important, AI processing on the endpoint is advantageous.
For advanced operations, there may be cost advantages in distributing the AI computations across the endpoints as opposed to doing them on the backend servers.
To manipulate audio and video streams on the server-side, the streams must be decoded at the server, appropriate AI algorithm or filter applied, the streams re-encoded, and then sent back out. This process can add some cost and delay.
If you have an end-to-end encrypted meeting, the video can’t be decrypted on the server, so if the AI is running on the server, none of the AI enhancements can be applied in an E2E encrypted meeting.
So, where should the AI run? In a recent session I moderated at an Enterprise Connect virtual event, panelists from Cisco, Microsoft, and Five9 discussed where AI in video solutions should be applied. The panelists had some consensus that AI will appear on both sides in the future, and which side it goes on will be based on what you are trying to accomplish. In my discussion with them, Pexip also indicated that in the future, there is likely to be some sort of combination of endpoint AI processing and server backend AI processing.
Will Nvidia Maxine Promote Market Disruption?
I think back to when Zoom launched, and few would have predicted the amazing success Zoom has achieved, now competing across all markets for both cloud-based video meetings and audio services. It clearly disrupted the market. One of the questions I asked Pexip, Cisco, and Nvidia was whether Maxine will enable a nimble, small competitor to enter the video communications market and disrupt it.
I asked this question because of the compelling capabilities Maxine offers. With Maxine, a company can enter the market without much development effort and could rival some of the video solutions out there today.
What Capabilities Does Maxine Offer?
Nvidia has created many AI algorithms that provide capabilities such as facial recognition, noise cancellation, speech recognition, video background blur or replacement, etc. Each of these pretrained algorithms is invoked through a software development kit (SDK). Sometimes the functionality in one AI model actual invokes functionality found in a different, but related AI model, so under the covers, there can be SDKs that actually call functions that are part of other SDKs.
Maxine is a set of three SDKs providing interfaces into Nvidia’s pretrained AI models for Video Effects, Augmented Reality, and Audio Effects. These have a robust set of capabilities as described below. Note, all images shown are courtesy of Nvidia.
1. Video Effects SDK
Super resolution enables up to four-times imaging scaling. It enhances details, sharpens output, preserves textural details of the video images. Look closely at the image below, and you can see some sharpening and enhanced clarity of the images going from left to right.
Face codec provides smooth full-motion video with up to ten-times bandwidth reduction. Face Codec sends a keyframe along with facial keypoints. The keyframe and these keypoints are run through a neural network to produce the output image. It allows transmission of only the keypoints and not an entire compressed image, which is what reduces the bandwidth so significantly.
Artifact reduction recognizes “image blemishes” and removes them. When video is compressed, some image areas may contain compression artifacts; this feature removes them.
Video noise removal enhances the image detail by removing low-light camera noise. Users with sub-optimal lighting typically have poor video quality.
Virtual background is used to remove a background and blur or replace it. This has become a standard feature across our industry, and some companies are looking at Maxine to do this for them.
2. Augmented Reality SDK
Face tracking detects human faces in images and videos and is a building block for virtual background and other facial recognition features.
Face landmark tracking recognizes facial features and contours, tracks head pose and facial deformation due to head movement and expression. This feature has a variety of purposes, including knowing if someone is paying attention and even for some emotion detection.
Face mesh creates a 3D mesh representation of a human face with 3,000 vertices. This could be used for facial authentication, or if a person wanted to use an avatar, face mesh would certainly enable some compelling graphical images.
Body pose estimation can be used for activity recognition, motion transfer, and virtual interactions. While perhaps not so useful for video conferencing, it can be used as an estimator of engagement. Maybe, it could be useful when holding long meetings, and the moderator asks everyone to stand up and stretch.
Eye contact aligns eye gaze with the camera. In the left side of the image below, the person’s eyes are looking away. Eye contact uses the other capabilities in the AR SDK to recognize eyes and facial features and manipulates the image to make it look like the person is looking at the camera. Microsoft also has this capability, although it is not likely built using Maxine.
Audio2Face animates an avatar or digital face based just on the audio input. Perhaps not useful for business video meetings today, but over the years we have heard how some people may want to be represented by an Avatar to avoid meeting fatigue.
3. Audio Effects SDK
Noise removal eliminates common background noises while preserving the speaker’s natural voice. Avaya is using this, and it is what Pexip is thinking about using, per the Pexip press release.
Room echo removal detects reverberations from an audio stream and eliminates or reduces echo.
The three Maxine SDKs can be combined with the DeepStream and VideoCodec SDKs to provide a processing pipeline including video decode, encode and the ability to handle multiple AI effects. The Jarvis SDK can also be used alongside Maxine to add language-based capabilities such as transcription and translation.
4. DeepStream SDK
Delivers an end-to-end streaming pipeline for AI-based, multi-sensor processing, video, audio and image understanding.
5. Video Codec SDK
It provides hardware-accelerated video encode on Windows and Linux devices.
6. Jarvis SDK
Speech recognition converts speech to text and allows users to search for keywords in closed captioning and text recordings.
Natural language is used to understand intent and context.
Sentiment detection can detect a person’s emotion. In most instances, this is based on text streams and on the audio. Although not specifically stated, I suspect Nvidia may be able to apply AI to a person’s facial expressions to detect sentiment.
Text-to-speech generates lifelike speech. Jarvis switches can be set to enhance the tone and expressiveness of the speech along with the option for different voices.
Real-time language translation can take spoken English and translate it into five languages: German, French, Russian, Spanish, and Japanese. More languages are also on the way.
Is Disruption Imminent?
One of the arguments against disruption made by the video communications vendors is the idea of best in class. Given the number of investments companies like Cisco, Microsoft, Pexip, Zoom, and others have put into developing their own algorithms for audio/video compression and processing, it may be difficult to imagine that Nvidia Maxine can offer the same A/V quality these companies can provide. Yet, one thing we are seeing is interest in Maxine for capabilities a video communications company may not have developed on its own.
We see in the AI universe that there is a lot of sharing going on. Given the massive impact machine learning is having in so many different fields, AI researchers are constantly developing new AI models and tweaking old ones to make them better. These are often shared within the broader AI community.
I can see a day in the not-too-distant future where Nvidia’s AI audio and video algorithms, even if not the best in class, will certainly be good enough in most video communications situations. Nvidia told me that they are not really thinking about a market disruption per se, rather they are providing Maxine’s SDKs and the Nvidia GPUs to a wide variety of markets and use cases. “The goal of Maxine is to provide the building blocks and tools for developers to implement the best workflow for their use case… Consumers are looking for improved video conferencing and live streaming experiences through features enabled by Maxine. These features require higher compute power, and Nvidia GPUs are much more efficient at high compute workloads. Nvidia is reducing costs to developers by offering pre-trained AI models and GPUs that can deploy simultaneous AI effects to millions of users concurrently,” a Nvidia representative said.
Given the array of functionality already available, Nvidia Maxine and Nvidia may provide the opportunity for a small, well-funded cloud-based startup to create a compelling video meeting service leveraging Maxine’s SDKs and the other AI capabilities available from Nvidia. What could result would be a video service that is good enough and at a lower price point than what we see in the market today.