Assessing Gen AI's Impact in the Contact Center, Part 2Assessing Gen AI's Impact in the Contact Center, Part 2
In this second part of No Jitter’s Conversations in Collaboration with Forrester senior analyst Christina McAllister, what’s at stake for every generative AI pilot is defined
February 16, 2024
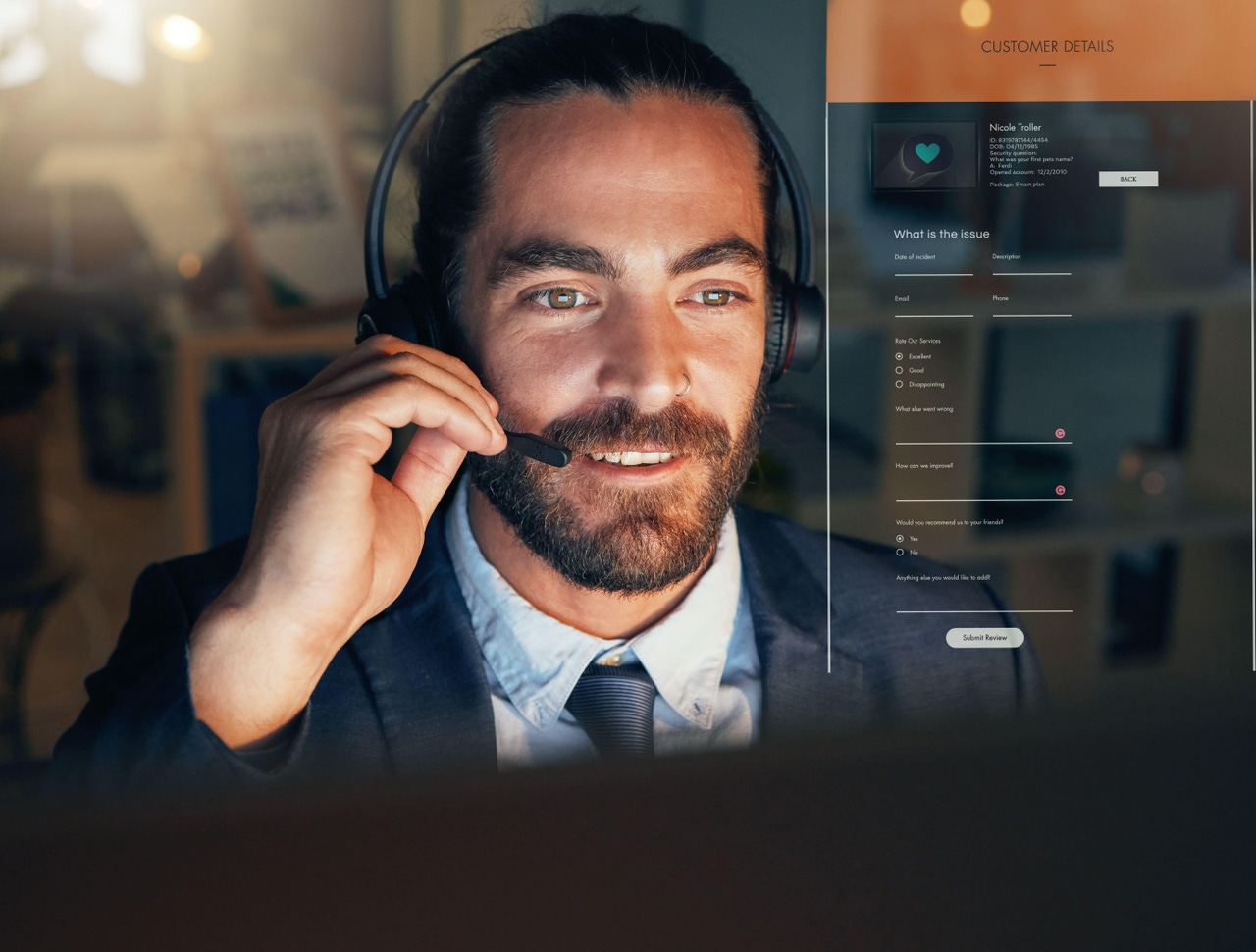
Welcome to No Jitter’s Conversations in Collaboration series. In this current series we’re asking industry leaders to talk about how AI can boost productivity – and how we define what productivity even is – with the goal of helping those charged with evaluating and/or implementing Gen AI to have a better sense of which technologies will best meet the needs of their organizations and customers.
This is part two of our conversation with Christina McAllister, a senior analyst at Forrester, who helps customer service and customer experience (CX) leaders transform their strategies and capabilities in the age of the customer McAllister’s research focuses on the technologies that enable and augment the customer service agent. These include customer service cloud platforms and applications, AI-infused agent workspaces, conversation intelligence, and digital engagement channels. Her research also explores how AI is transforming contact center operations and the agent experience.
In this installment of our conversation, McAllister covers AI as a way to optimize quality monitoring, then dives into the math that will justify the cost of using generative AI in the contact center.
![]() Christina McAllister, Forrester |
NJ: Let’s talk about another way we’re seeing generative AI being used in the contact center – AI being used to scan all of the interactions versus the supervisor only reviewing the ones that are flagged or an otherwise small percentage.
Christina McAllister (CM): In the average, non-AI-enabled “traditional” call center, approximately 2% of an agent's calls, chats or interactions are evaluated by a quality [auditor or assurance] person or supervisor. Two percent is woefully low – a very bad ratio.
There are solutions, often called automated quality monitoring, that can auto score multiple [metrics] – usually along the lines of “met or not met” to get at those broader behaviors.
The value there is in having a look at your agents’ performance trends over time rather than blips in a month. It helps supervisors understand the difference between agents just having a bad day versus a sequence of bad days versus a trend or behavior they need support or to improve [agent] skills in certain areas. In those [use cases], I am not seeing a ton of gen AI applied to the actual analysis necessarily. I am seeing it used to generate recommendations or summaries of what that agent needs support with, or summaries of key issues or “bright spots.”
Another piece I have seen is when a supervisor is provided with [a gen AI-produced] summary of an interaction that was already generated for the agent [after the call]. Basically, this approach borrows that summary and reuses it.
This is basically creating structured data out of unstructured data by providing answers to questions like “What was the outcome?” or “What were the agent steps?”. [This allows] the supervisor to see at a glance what happened on that call – and then dig into the areas they want to.
It's a slightly harder to attribute value there simply because the way people measure the efficiency of their QA function is really variable. If done well, the value would in reducing the time to evaluate, so that more calls could be evaluated. or more calls could be evaluated, or [the same number of] calls with fewer people.
When it comes to the summaries and how they're applied to the call monitoring process, the application would be to help whoever's looking at them to understand at a glance what's going on. They could also get contextual recommendations around where they should focus their time because their time is split across all the agents they support. Giving supervisors the ammunition to coach better is a priority for most of the buyers that I talk to.
NJ: How does cost factor into the analysis on the transcript?
CM: Well, if you were already summarizing every interaction [with gen AI], then you’re just borrowing that summary and placing it in multiple locations. That's one way to [reduce queries to the model].
You could summarize the transcript and have it built into actions like I described above, but the problem with the real time piece is that it's on every turn of the conversation. [Meaning that] every time I say something to you and you say something back, I'm making a new hit on the model. The conversation is not as long, but it [requires] constant “hits” on the model, whereas it’s one hit on one entire transcript. That's not inexpensive, but it's not as frequent.
NJ: How does the enterprise go about evaluating the cost of investing in the summarization versus the benefit they receive on the other end?
CM: I have not seen many in-market examples of generative AI. I mostly see folks automating the quality flow with the traditional kind of conversation analytics. That is a huge value savings already. Some companies have quality analysts that are evaluating two percent of the interactions. That person spends a lot of time just listening to the calls. So the [analytics] are changing the balance of effort across the across the scope of that work. However, I don't see much adoption of the [gen AI use case] I mentioned. I think that will come, but for the enterprises I’ve talked to its not a high priority.
More broadly, I honestly don't think that buyers are educated enough on that cost challenge of [gen AI]. I think that that will come [since] I'm seeing many pilots open up where they're experimenting and starting to look at how much it will cost in the end.
I don't know that all the vendors have landed on their pricing strategy for their gen AI features. They may be working at a loss for now, but they're not going to be able to do that forever, especially if they're leveraging a third-party model that they owe money to – like if they need to pay OpenAI for access to their API.
If the cost of ownership of a solution that uses generative AI stays constant, there's a level of diminishing returns where you're not going to be able to crunch an agent's efficiency any lower than a certain point. But, you'll still be paying that fee to the generative model.
So [if that’s the case], where is the split going to happen where customers say, hey, we didn't need generative for that, or we need to start anchoring these [responses] so that we're not continuing to pay every time that we say exactly the same thing.
That's where I'm starting to do the math and the math “doesn't math” for me. Having built a lot of these business cases earlier in my career, the math is not going to math for long, I think. So, it'll be just a matter of time until the vendors are forced to have a strategy that blends both approaches where not everything needs to be generative because it's expensive for everything to be generative.
The biggest questions I get from Forrester clients who are cost-centric are: Is the ROI really there? How expensive is this going to be? The answer is a very big “it depends” because the vendors haven't really landed on their mature pricing strategy for this. We're still in learn mode across the market.
NJ: Do you think learn mode persists through 2024? What does your crystal ball tell you?
CM: I think for certain use cases like summarizations, we will land on something that we feel comfortable with and that the cost – as long as it balances with the cost of not spending that time [and/or] the downstream value of using that summary in different ways.
When it starts coming into real time use cases where you need low latency, high accuracy, [and/or] low hallucination, that’s where I haven't seen that settle yet. Most mature enterprises would consider that an experimental use case.
A lot of folks are buying gen AI as more of an innovation approach. They're thinking about it as if they’re doing something with emerging technology. It's not necessarily a ROI play for some big enterprises. But, for some of them, it will be [an ROI play].
I think we're going to see a lot of pilots in 2024. But for some, those pilots are not going to convert because the math doesn't make sense. I expect to see a lot of pilot churn for some of the agent-facing real-time use cases. There will be some wins, but I don’t think it's going to be a slam dunk for some of the vendors that have not thought through the long-term mechanics.
Want to know more?
On the point McAllister made regarding not everything needing to be generative AI because it’s expensive, check out NJ’s interview with Ellen Loeshelle of Qualtrics. Also see Frances Horner’s article on how AI can be used to assist in performance coaching. This article by analyst and frequent NJ contributor Sheila McGee-Smith discusses how Amazon Connect integrated generative AI into its Wisdom offering via Amazon Q.
About the Author
You May Also Like