Afiniti Taps AI to Optimize Customer-Agent PairingAfiniti Taps AI to Optimize Customer-Agent Pairing
Artificial intelligence can significantly improve a contact center's business performance.
December 18, 2017
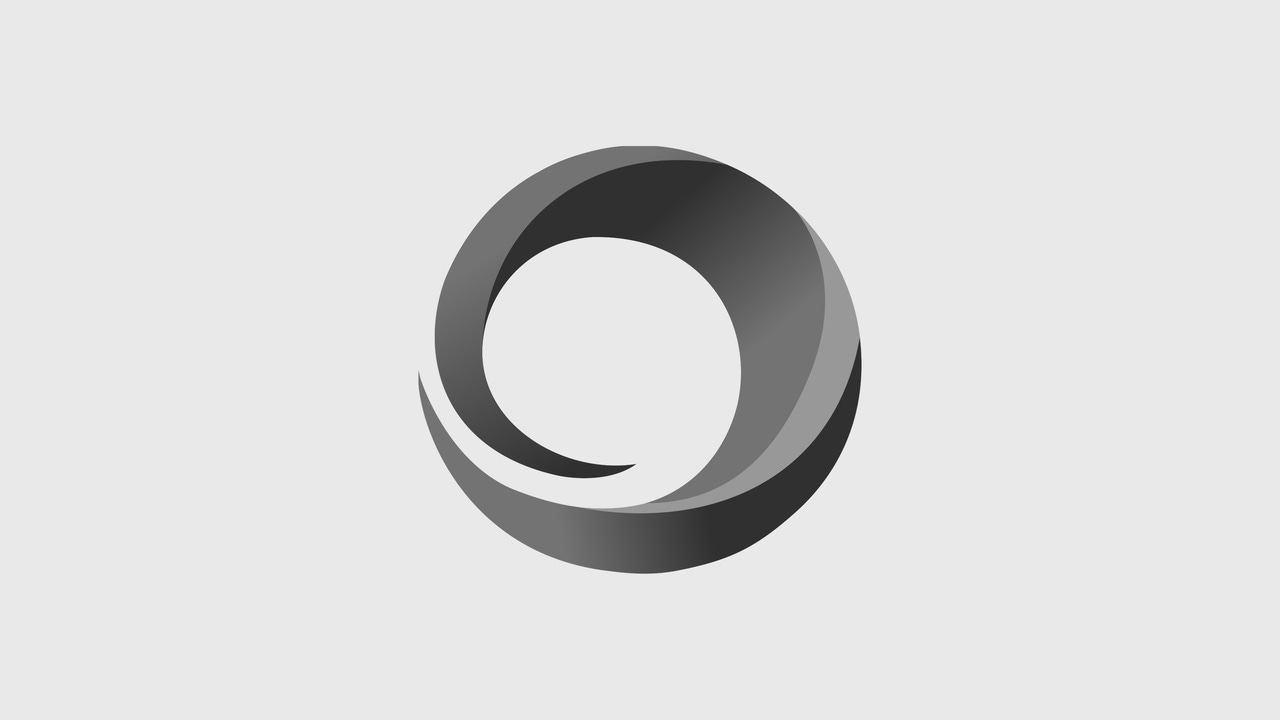
Afiniti is one of the companies leading the way in applying artificial intelligence (AI) and machine learning technology to the contact center. The company has developed AI-based technology that pairs contact center agents with customers to help measurably improve contact center business performance. Afiniti is a partner in Avaya's A.I.Connect initiative, but the company's solutions work with any contact center or telephony switch.
Afiniti is a passionate believer that the contact center should be in the service of an organization's bottom line. The core function of pairing customers to agents should support this premise by routing customers to the agents most likely to achieve the desired outcome, whether it be new sales, upselling, retention, or problem resolution. The company's AI algorithms enable intelligent routing for the optimization of any of these desired outcomes.
The idea of pairing the agents most likely to achieve a desired outcome with customers is not a new concept; tracking and monitoring agent performance is standard contact center procedure. What's unique about Afiniti's approach is how it improves the overall performance of the entire contact center, not just high performers. Plus, its effectiveness can be accurately measured. Afiniti is so confident of its technology that it is willing to install its solutions risk free and at no cost to the customer, taking payment based on actual performance improvements.
How Afiniti's Solution Works
Afiniti's technology uses several supervised machine learning techniques, including regression and classification analysis along with Bayesian analytics and heuristics. Using regression analysis, Afiniti's system matches customer characteristics and behavior with complementary agent characteristics and performance to estimate a probability of how likely a particular agent will achieve the desired business outcome with a particular customer.
While this sounds simple and straightforward, it's really quite complex. The graphic below shows a simplistic overview of the logic flow when pairing agents with customers.

Afiniti's "customer agent" pairing algorithm uses AI machine learning to optimize contact center performance.
It all starts with a customer's automatic number identification or caller ID from the IVR system. Using the customer's phone number as an initial data key, Afiniti's system begins accessing information from more than 100 public and private data sources using a data-chaining mechanism. For example, from the phone number, Afiniti can find out a person's name, street address, city, and state. From this location information, the company can access census data to determine characteristics of the person's neighborhood, city, or town. It can then pull down an individual's data from credit reporting bureaus, companies that track online user behavior, social networking sites, and even organizations that track purchasing information.
All of this customer attribute data feeds into a neural network to compute customer types or customer segmentations, a process that is accomplished in a matter of microseconds. Companies that use Afiniti's system will normally have a small number of customer types, each of which may be further segmented into subtypes. Having more than 100 different customer subtypes within one queue isn't unusual for a company.
We should note many end user organizations already have their own customer segmentation schemes. Afiniti will use an organization's own segmentation types as inputs to the neural network, along with all of the other data Afiniti gathers about a particular customer to generate a unique Afiniti segmentation.
The process of matching an agent with a customer is done by a machine learning technique called regression analysis. The Afiniti system feeds the regression algorithm with data attributes from both the customer and the agents currently on duty. Customer data includes the customer type along with any other existing data from the CRM database. IVR digits and queue data play into the analysis as well, because they provide information on customer intent -- the reasons for their calls. Do they want to buy something or speak with tech support, for example, or do they have a request to discontinue service? Agent attributes, including performance, also feed into the regression model. Other attributes the model uses are based on an optional 20-minute survey administered to new contact center agents or upon initial deployment of the Afiniti solution. These other attributes can include a wide variety of factors such as likes/dislikes, married/single, male/female, children/no children, hobbies/interests, athletic or not, music interests, sports interests, and where they live.
Based on the customer and agent data, the Afiniti software uses the AI regression model parameters to compute how likely a particular agent is to meet the business objective (sell, subscribe, upsell, retain, etc.) successfully. These computed agent probabilities are compared to one another in terms of the differences between them (this is represented by the ∆s [deltas] in the graphic).
Finally, based on contact center service level commitments and operating rules, the Afiniti software makes an optimal agent pairing. It follows all contact center rules in doing so: length of time in queue, equal call distribution among agents, etc.
Importantly, Afiniti's solution works within the constraints of the contact center's operating rules, and it doesn't necessarily favor one agent over another. In the high-volume contact centers where Afiniti operates, every agent is purposed where he or she can best satisfy the company's business objectives. And, because of how Afiniti measures its own performance, every agent is treated fairly.
Click to Page 2: Training and Continual Learning, and more
Continued from Page 1
Training and Continual Learning
The Afiniti software takes from two weeks up to four months to train itself on agent performance after deployment (and it's always deployed on premises for privacy and security reasons). It then goes into production.
The self-training may seem like a long time, but consider that a single contact center agent may take about 700 calls/month, or approximately 2,800 calls over a four-month period. If a company has designated 100 different customer types, then that equates to 28 calls per customer type, which is really not a lot of data with which to train a machine learning system.
Consequently, once the system goes into production, daily agent performance continuously feeds into the regression engine, which in turn updates the system for the next day's calls. This continual learning mechanism is useful because agent performance and customer preferences may change over time, and events that temporarily skew the need for certain types of agents might pop up.
The constant feedback loop also helps train the system for new agents. The new agent's attributes are entered into the system immediately upon hiring, and the Afiniti software instantly begins computing that agent's likelihood of success when considering optimal pairings. Of course, with time and experience, that agent's pairing performance should improve.
Proving Afiniti's Own Performance: How Afiniti Makes Money
As I mentioned earlier, Afiniti is so confident of its technology that it will install its AI-based agent-customer pairing system in a contact center at no charge. The customer and Afiniti agree in advance on the system's performance measures, and Afiniti receives payment based on what it proves its effectiveness really is. For example, if an Afiniti customer wants higher sales conversion rates, then Afiniti gets paid based on some percentage of the higher sales numbers for which it can prove responsibility.
Afiniti proves its own performance using statistical sampling: every hour, the Afiniti system turns off customer-agent pairings for a given amount of time. It then compares agent performance while the Afiniti system is turned off with agent performance when the Afiniti system is turned on. In this way, both Afiniti and the customer can see the impact Afiniti's solution is having on the desired outcomes, and base payment on actual measured system performance.
Proof That It Works
In a case study on the Afiniti website, T-Mobile describes how it turned to Afiniti's solution when seeking a higher conversion rate for prospect to paying customer. According to T-Mobile, Afiniti's system is responsible for $70 million in additional new sales annually. In additional case studies, other multibillion-dollar companies show sales increases as percentages as opposed to dollar values. Nevertheless, in a large company, a small increase in sales conversation amounts to a lot of money.
Afiniti targets its software toward large, high-volume contact centers. The company claims that all of the major U.S. telcos use its technology and that it presently has 150 deployments.
CB Insights, a tech market intelligent platform provider, recently ranked Afiniti among the 100 most promising private AI companies in the world. And, based on its last round of funding, Afiniti is worth approximately $1.6 billion.
AI is clearly moving into the communications and collaboration domain, whether to add intelligence to contact center routing; provide intelligent chat bots and virtual digital assistants; or start meetings based on facial recognition. Afiniti's story resonates because it has real products in production using AI/machine learning, and its revenue model is so transparent and straightforward.
To learn more about how AI is being used in business communications, consider attending the session at Enterprise Connect 2018 titled "Understanding AI--Beyond the Hype." Enterprise Connect, will take place March 12 to 15, in Orlando, Fla. And, if you register now using the code NOJITTER, you can save an additional $200 off the Advance Rate or get a free Expo Plus pass.
Related content:
Artificial Intelligence -- No So Artificial