The Adaptive Nature of NetflixThe Adaptive Nature of Netflix
As more enterprise environments rely on streaming content, delivery mechanisms will have to strive to achieve Netflix's adaptive delivery model.
February 23, 2012
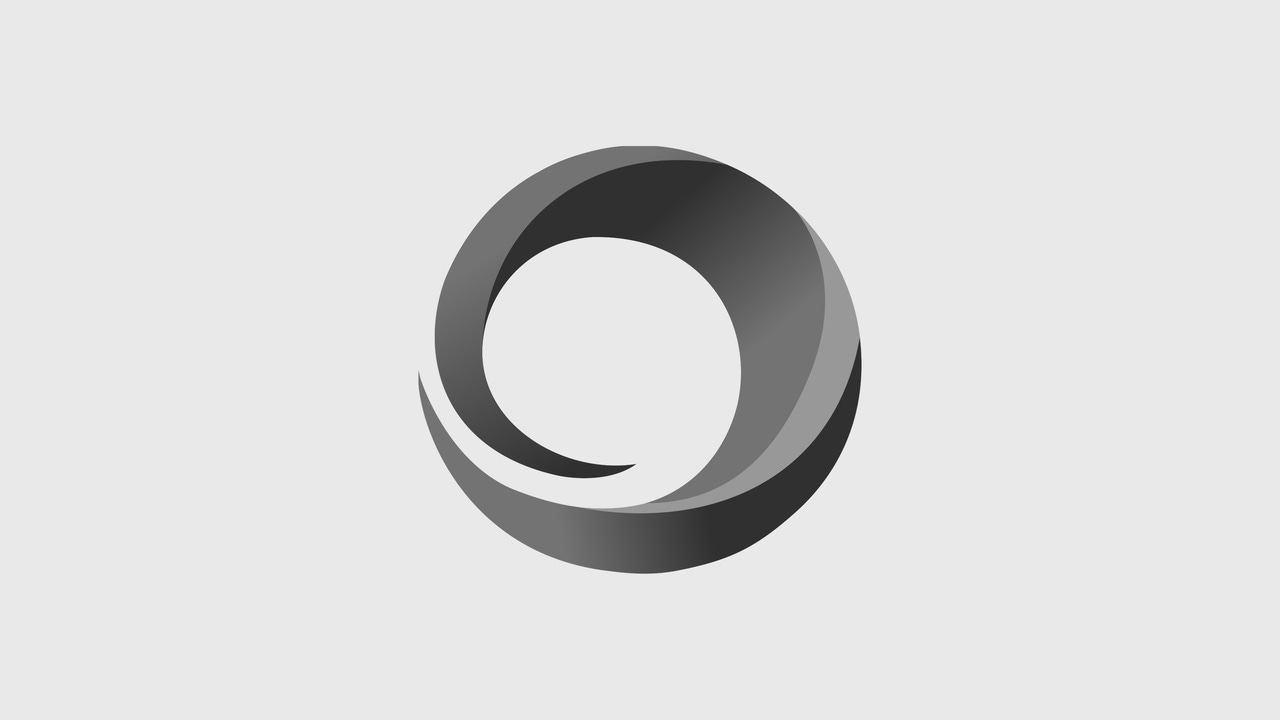
As more enterprise environments rely on streaming content, delivery mechanisms will have to strive to achieve Netflix's adaptive delivery model.
As a leading provider of media content, Netflix has established a reputation as an easy-to-use, fast, and reliable provider of movies. Initially, movies were delivered and returned using the US Mail. However, with the increasing popularity and prevalence of streaming, Netflix did an excellent job of adapting its service model. In this article I am going to focus on Netflix's ability to deliver streaming content to different types of devices and how that content impacts the available network capacity.
Before diving into the test results, it is important to touch upon something that may seem obvious, but is worth noting. The viewing experience on different devices is going to vary, which in turn poses a challenge to Netflix. I would expect that the picture quality on a Samsung HD TV connected to a Blu Ray player would be better than the picture quality on a MacBook or iPad. If Netflix is going to be a successful provider of streaming content then they have to consistently deliver a smooth, uninterrupted viewing experience regardless of the output device and underlying network.
On to the tests. Testing consisted of several components. The ISP was Verizon FIOS, which provided 25Mbps in both the up and down direction. Three devices were tested--a Samsung Blu Ray player connected to the network at 100Mbps, an Apple MacBook connected to the network at 1Gbps, and an iPad which was connected to the network using 802.11n. A variety of HD movies was selected, each of which was viewed for 15 minutes on each device.
To no great surprise, all of the movies were successfully streamed; each device played the movies very nicely without any glitches. However, the real story is what was discovered under the covers (on the wire).
The first aspect analyzed was the average amount of bandwidth used in the downward direction by each device. The Samsung Blu Ray player on average used 3.1Mbps; the iPad on average used 1.3Mbps; and the MacBook on average used 4Mbps. After digging into the data deeper, a more interesting finding was discovered. Netflix dynamically adapts its service by evaluating several factors before determining how much bandwidth should be allocated in order to deliver the best quality picture.
The factors that come into Netflix's decision are a) determining how much bandwidth a device is capable of consuming; b) determining what type of output quality is required by the requesting device, i.e., a large HD TV versus a small handheld tablet; and c) determining the actual data throughput a device's connection is capable of sustaining. The following table summarizes the testing results.
Available Bandwidth Utilized = Average Download Data Rate/Peak Date Rate Achieved
The key point here is that even though one device is capable of consuming data at a higher rate than another device, Netflix does not indiscriminately consume all of the available bandwidth. It also factors in what type of device is being used to display the content. The above results show that Netflix utilizes a much higher percentage of available bandwidth for the Blu Ray (42%) versus MacBook (20%) or iPad (16%) since the Blu Ray is required to deliver streaming content to a 55" HD TV. This is a testament to Netflix's ability to adapt to different network connections and devices.
As more and more environments rely on streaming content, such as distant learning institutions and training within enterprises, delivery mechanisms will have to strive to achieve Netflix's adaptive delivery model in order provide successful streaming services.
In future articles I plan to share the results of additional types of testing and look at the actual amount of data that Netflix and other on-line service providers actually consume.