Elevators vs. Networks: Applying Predictive AnalyticsElevators vs. Networks: Applying Predictive Analytics
Can you predict a network failure?
August 4, 2017
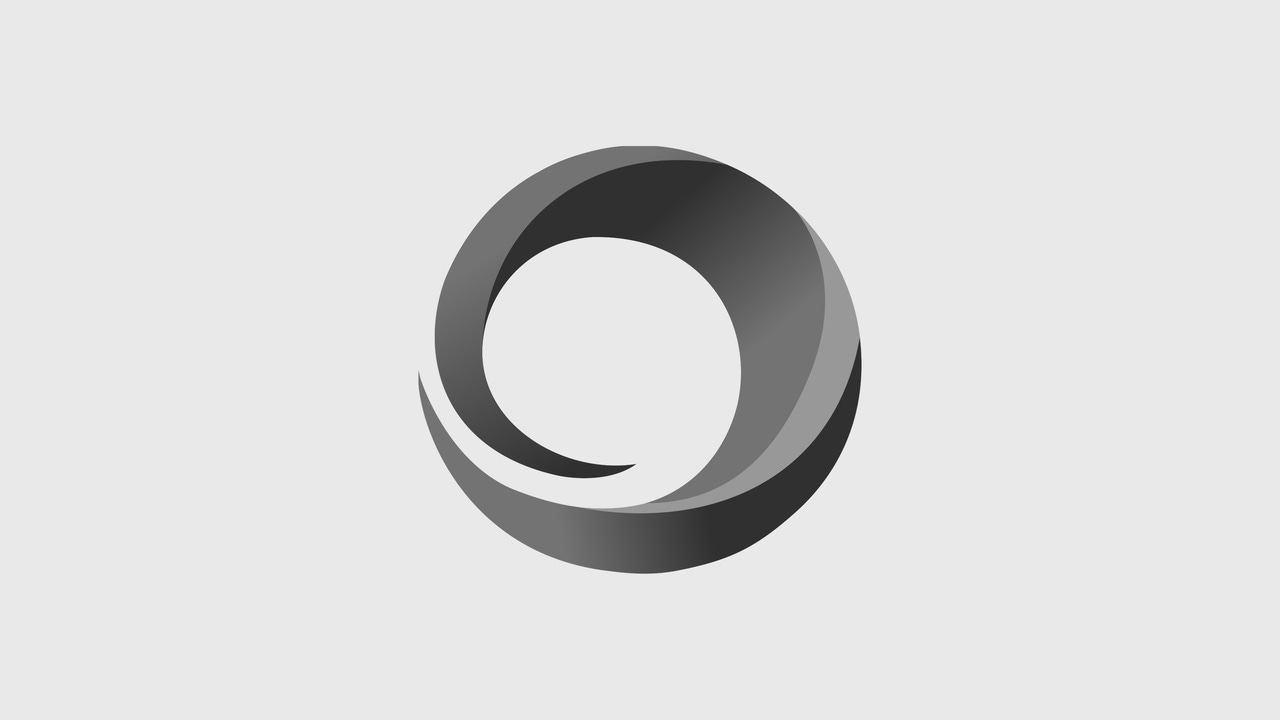
I was recently watching a television ad that made me think of how predictive analytics can be applied to networks. The ad showed a repairman entering an office building and saying, "I'm here to fix the elevator." The security guard responds, "We have no problem." Then the repairman replies that the problem will occur in three days, and he was able to know this through access to IBM Watson performing predictive analysis.
In regard to networks, could predictive analytics allow us to predict network problems such as failures, degradation, and performance issues? It seems a reasonable question to ask, however, it requires some knowledge of analytics technologies to understand the success of predictive analytics for networks.
The Elevator vs. the Network
The elevator is part of the building. It has its own architecture and infrastructure. Most of what happens to the elevator as it goes up and down is repeated every day. The elevator's environment does not change much unless there is a fire, earthquake, or some other factor which affects the elevator's operation. There is a long history of the elevator operations within the building. There is even a greater history of that same type of elevator operating in other buildings available to the elevator manufacturer.
The network is a different story. The network may change based on redesign, traffic behavior, routing changes, application changes, and software modifications. The network is not as static in its operation when compared to the elevator.
Service providers are trying to implement Network Function Virtualization (NFV) and Software-Defined Networks (SDN), which change provisioning, billing, and operations. One of the biggest changes will be the ability for an enterprise to demand network and capacity changes to be delivered in minutes rather than weeks. This makes it harder to predict network operation because the data collected is about a dynamic (not static) network. There may be very little historical data available after the customer makes changes to the network's operations. Less data means weaker predictions.
Predictive Analytics Foundation
Predictive analytics incorporates machine learning (ML) and artificial intelligence (AI) processes. AI can be implemented in any device that measures its environment (network), and takes actions that maximize its chance of success for a goal such as predicting network operation. AI is applied when a machine mimics human functions such as learning and problem solving. ML gives computers the ability to learn from data without being explicitly programmed in advance.
Predictive analytics has improved, benefiting from advances in AI and related fields. IBM's Watson is an example of this implementation process. The limitation of AI is that the accuracy of the predictions will depend on the quality the data collected.
Predicting Network Performance
The application of forecasting algorithms can help manage network resources. Deep learning can be a useful tool for network performance and quality optimization. Deep learning is part of machine learning based on learning data representations, as opposed to task-specific algorithms. Deep learning architectures can produce results comparable to and sometimes superior to human experts. An example would be to preemptively rebalance the traffic load on the network and provisioning more capacity in real time.
Predictive analysis can also examine data traffic trends and patterns based on usage types to provide early warnings of possible issues. This means that a good predictive analysis tool can predict the impact of changes and avoid such impact.
Proactive Security Implementation
Security attacks have become more sophisticated. All the tools that have been deployed work, but attackers have learned ways to circumvent the systems and software used to produce security. Predictive analytics can be used to rapidly detect security breaches. It could also provide clues and alerts to variations in human behavior that are suspicious.
Is Predictive Analysis Worth It?
At this time, predictive analytics for networks needs to mature. If the enterprise has a lot of relatively static network design, predictive analytics will probably work and serve a benefit. But once we introduce NFV and SDN, the enterprise will be operating a network that has little or no history. Assuming that the enterprise continually makes changes to the network capacity and implementation based on evolving business requirements, there may never be enough history cumulated to allow the performance of adequate predictive analytics.
On the other hand, service providers who own the infrastructure providing the services should have a much better view of the overall network. They own and operate all the facilities and they can collect data that has historical significance and be applied for predictive analytics. The providers can then deliver better SLAs, not just service level goals. (See, "Voice & Video Performance SLAs: Do Yours Deliver?" and "Know Your SLAs.")
Remember that AI and ML results are only as good as the data input. Don't assume that the results are valid until verified. Since data changes over time, you will have to periodically revalidate predictive analytics results.