AI Plus Speech Equals New ValueAI Plus Speech Equals New Value
Conversational AI is going to drive new workplace value for voice that we couldn’t even imagine a few short years ago.
April 8, 2019
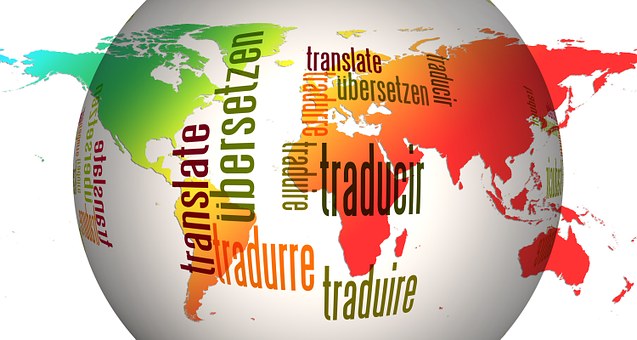
By now, you’ve probably had your fill of Enterprise Connect reviews, here on No Jitter and elsewhere, and given how impressive the conference was, the extensive coverage has been warranted. EC19’s moment has largely passed now, but I’ve got a takeaway here you’re not going to see anywhere else, and it’s only loosely tied to the event.
Speech technology was one of the more interesting themes from EC19 -- and not just because I spoke about it -- and if you’re wondering what the buzz is about, I’ve got two different examples to share that came out the research I did for my talk.
Like anything else, this topic is only interesting when thinking about it in a certain way. I opened my talk by explaining how artificial intelligence (AI) and speech are two distinct topics on very different trajectories. AI is super-hyped, all-consuming, and moving in all directions at the same time. There’s no center of gravity, and every vendor is trying to AI-infuse or AI-enable whatever it’s selling. Some of these efforts will bear fruit and some will quietly go away -- and speech is one of the applications in AI’s orbit.
Speech technology, on the other hand, is pretty mature, and to date has mostly been utilitarian, with use cases related to audio transcription and language translation. Now, picture a Venn diagram and the overlapping space between the two, and that’s where I see potential for new value. AI is a new twist on speech recognition, and for all kinds of reasons, it’s taking things to a whole new level.
Aside from making incremental but very noticeable improvements in speech accuracy, AI brings context, intent, sentiment, etc. to the equation, and that elevates the value of speech -- and voice, really -- for use cases like collaboration. This is a separate topic altogether, and for this post I just want to illustrate what’s happening with two specific examples I featured in my talk.
Otter.ai -- Seeing is Believing -- Real-time Transcription
I’ve cited this example previously, but it also works well for this post. Otter.ai, a standalone offering from AISense, is a leading example of real-time transcription offerings that I think will soon become a standard feature for collaboration platforms. Regular transcription is after the fact, but real-time is in the moment, and is emerging as a way to make meetings more inclusive.
Aside from not having to take notes -- and thus be more engaged during a meeting -- this helps participants who are hearing-impaired or can’t follow English speech all that well keep pace with everyone else. Think about meetings with multicultural participants where English isn’t native, but also think about speakers with strong accents that even English-speaking participants have a hard time following.
I’m being cheeky, but what comes to mind here is this scene in Austin Powers when he’s blathering on with his Dad in cockney patois. Not only is the cockney so thick that even English-speaking people need subtitles, but there’s the added layer of decoding the slang -- and that’s yet another AI problem that I’m sure the folks at Otter are hard at work on.
Speaking of decoding slang and keeping you smiling, I’d be remiss to not lay on the camp even thicker with this you-can’t-get-away-with-stuff-like-this-any-more encounter from Airplane, a scene that no doubt inspired Mike Myers when talking naughty with Michael Caine in Austin Powers. Cut me some slack, Jack, it’s still funny.
Coming back to the collaboration environment, the combination of real-time transcription and real-time translation creates another compelling use case. Variations of this have been around a for a while, and we saw a great example of this during Microsoft’s EC19 keynote. Individually, each of these capabilities is impressive, but when you show them working in tandem -- as Microsoft did with a Chinese speaker having her speech translated to English simultaneously -- it’s pretty magical. (Watch the keynote video below, at the 15:28-minute mark).
Then there’s the AI part, and this is where a lot of new value will come from. Otter’s Teams application allows for speaker tagging, and with all the text being searchable, it’s easy to find all the spots where one person speaks, and even those where two particular people are speaking to each other, or add a search word to find out whenever that word occurs in the transcription is being discussed. The search possibilities are endless, and this makes the transcription a powerful value-add to meetings.
Other important features include customizing language references so the transcription engine will accurately track specific terms or acronyms for your industry or particular project. Otter.ai integrates with most of the major collaboration platforms, so it’s a value-add for what you’re already using. There’s also two-factor authentication to ensure security for your workspace, especially for those joining a meeting remotely where their identity is harder to ascertain.
These features are pretty cool, but none of it really matters unless the transcription accuracy is there -- not just for reading, but also for real-time when you’re actually paying the most attention. Accuracy is a point of pride for Otter.ai -- as it is for every speech-to-text player I’ve been talking to -- and if you check out the team’s background, the pedigree is certainly there.
There’s more to the story, but let’s get right to the seeing-is-believing part. When you open this link, you’ll be able to view Otter’s real-time transcription, where you can hear the audio of me talking, along with the text of my speech appearing in real time -- with each word being highlighted in blue as its spoken and transcribed as it goes along.
Follow the bouncing ball, and as you’ll see, the speech-to-text is very accurate. The clip is about 1.5 minutes, so it’s not a long demo. For context, this is a segment during my talk at Enterprise Connect – talking about Otter -- and was recorded on a mobile phone about 20 feet away from me. All of this was done by Mari Mineta Clapp, who handles marketing on behalf of Otter, so a big thank you to Mari. These were hardly ideal recording conditions, but even with that, I think you’ll agree that the quality is good enough for enterprise collaboration purposes.
Click below to continue to next page: Google WaveNet -- Hearing Is Believing, and more
Google WaveNet -- Hearing Is Believing
You don’t need me to tell you about Google, but you might need me to tell you about WaveNet. Google strands run through every thread of the AI tapestry, and it’s not much different for voice. There’s a separate post to be written about that, but for now, all roads lead to WaveNet.
If you don’t know, Google made a savvy acquisition of U.K.-based DeepMind in 2014. This is the company that used AI to defeat the champs at Go, and if you thought IBM Watson was impressive for beating Ken Jennings, you need an AI refresh. The Go story is a topic for yet another post, and it’s an ominous sign of what’s to come as neural networks and deep learning find their way into everyday life.
I digress, but this takes us to WaveNet. I’m not an expert on how they do it, but WaveNets are based on neural networks that have developed new models of generating audio that are more accurate and natural-sounding than other text-to-speech (TTS) models. That’s all I’m going to say, and will now let your ears do the testing.
Below are two 30-second clips created by a Google team headed by Dan Aharon, product manager of cloud and speech products. During the course of my research for my Enterprise Connect talk, we discussed ways to illustrate how good Google’s speech technology has become. While the Otter example is about speech-to-text (STT) and real-time transcription, WaveNet is about using AI to create text-to-speech outputs that sound really human-like. Aside from getting the language right, the bigger challenge is generating utterances that have the natural flow, cadence, pacing, tone, etc. of the human voice.
Dan asked me to write a narrative to explain this, and that he would generate two speech samples for comparison. The first sample below is what’s called Standard TTS, and it sounds OK, but rather stilted. Compare that to the second sample, which uses the same narrative, but generated from WaveNet. Just to be clear, pay more attention to the audio quality than what’s being said.
The narrative is exactly the same for both samples, and it may sound confusing since it’s referring to the sound quality being different for each sample, but you don’t actually hear it within each sample. The first sample is one approach for TTS end-to-end, and the second example is for the second approach -- I realize it’s a bit awkward when listening to the narrative, but that’s just the way it turned out.
What really matters is the audio quality comparison, so that aside, I’m not going to say it’s a perfect emulation of human speech, but the WaveNet version is warmer and more natural sounding for sure, and from there, it’s not a big leap to see how this quality of TTS will make conversational AI second nature before long.
Google TTS -- Standard Example (without WaveNet)
Google TTS – WaveNet Example
TTS has a different set of collaboration use cases from STT, and the starting point is producing audio that sounds like we do, especially for listening to longer-form content such as a podcast that AI compiles for you based on excerpts from a long report you don’t have time to read, but can digest during your drive into work. Once you get comfortable with TTS applications, it’s not such a big leap to conversational AI, along the lines of what Google showed with Duplex last year. Despite the Duplex demo passing the Turing Test -- which definitely cuts both ways -- Google has some challenges to work through there, and like it or not, Amazon Web Services’ Alexa for Business is going to find its legs. At some point soon, conversational AI is going to drive new workplace value for voice that we couldn’t even imagine a few short years ago. Whether or not you believe what you’re seeing and hearing in this post, it’s here now, and I have no doubt the Venn diagram circles for AI and speech are going to move a lot closer together, and that’s going to be good news for collaboration. BCStrategies is an industry resource for enterprises, vendors, system integrators, and anyone interested in the growing business communications arena. A supplier of objective information on business communications, BCStrategies is supported by an alliance of leading communication industry advisors, analysts, and consultants who have worked in the various segments of the dynamic business communications market.