Conversations in Collaboration: ServiceNow’s Terence Chesire on AI, Automation and Defining Business ProcessesConversations in Collaboration: ServiceNow’s Terence Chesire on AI, Automation and Defining Business Processes
ServiceNow’s Chesire on how the ServiceNow platform can be used to define business processes, continually improve on them and, of course, how generative AI can be leveraged to add further efficiencies.
October 18, 2023
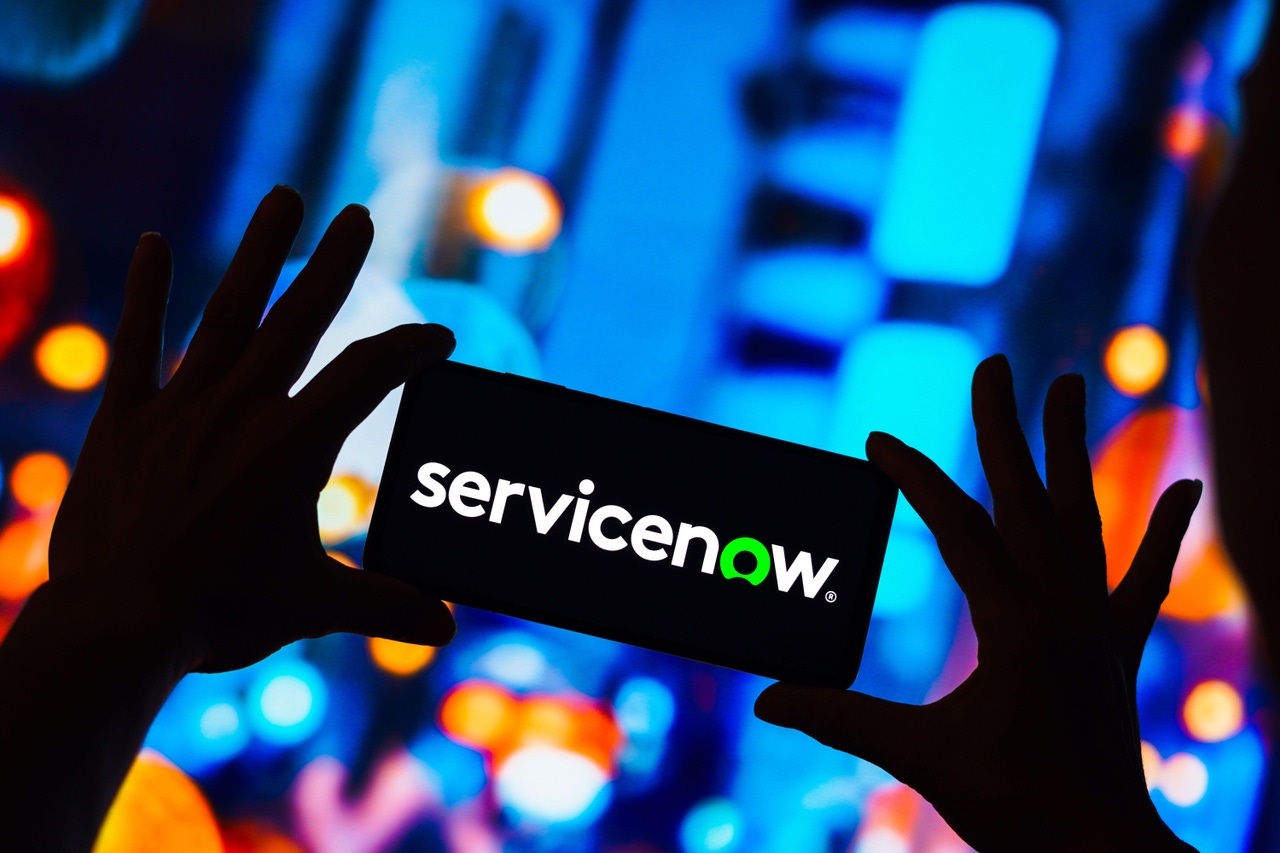
Welcome to No Jitter's ongoing series, Conversations in Collaboration, in which we speak with executives and thought leaders about the key trends across the full stack of enterprise communications technologies. Check out the links at the end of this article for all of the previous conversations.
NJ spoke with Terence Chesire, vice president of product management for customer workflows and industry products at ServiceNow. He is responsible for product launches and enablement, pricing and packaging, and technology ecosystem, in addition to supporting overall product strategy. Prior to ServiceNow, Terence served as VP of product management for BMC Software, where he launched their Helix product line, and SAP, where he launched their Service Cloud product line.
| Terence Chesire |
NJ spoke with Chesire prior to ServiceNow’s mid-September 2023 media briefing about ServiceNow’s Vancouver release (covered here in No Jitter Roll) during which the company announced generative AI capabilities for its platform and branded them as “Now Assist” which are available across multiple ServiceNow products including IT Service Management (ITSM), HR Service Delivery (HRSD) and Customer Service Management (CSM).
(Editor's Note: Artificial intelligence has a lot of specific, descriptive terms. Download our handy guide to AI vocabulary here.)
No Jitter (NJ): Can we start with a brief synopsis of ServiceNow and where your division fits within the organization?
Terence Chesire: You may have heard us described as the company that helps get work done. That simply means we help orchestrate tasks across an organization with our software. Most folks will be aware that their service desks utilize ServiceNow. We expanded it to HR – think of all the things you need to line up to onboard an employee from getting their security badge, to their laptop, to access to systems, etc. But I focus on customer service.
We think that while the market has been well served in the “front office” with [various solutions], sometimes things need to span the middle and the back office. For example, let’s say you initiate a bank transfer and it goes to the wrong account. Obviously, you’re very concerned and you contact the customer service team – you're really not interested in the phone call [per se], you're interested in how fast your funds can be refunded back to you.
NJ: Okay, where is ServiceNow in that example?
Chesire: The voice call goes into the contact center solution, but the minute they need to do something on their computer – verifying who you are, your account number – that is our software.
To give you something more tangible – Lloyds Bank is the example I'm bringing to mind.
What we did through workflow was automate about 91% of the exceptions so that while [the process] started with phone calls as [Lloyds Bank] continuously improved, they were able to get to a point where as the customer taps on their phone and says, “I think I sent this payment to the wrong person,” then that goes through a series of rules and they get reimbursed within seconds.
To do all that we had to integrate into dozens of systems. CCaaS was just one of them. Lloyd’s had many legacy banking systems that were third-party systems and [then they had] communications with other banks. So, integration is one of the core concepts, but we also had to orchestrate work [within Lloyd’s and across other third-party banks]. We refer to it sometimes as a system of action, which is executing a series of tasks to complete and resolve the issue.
NJ: To go a little deeper, it sounds like Lloyd’s already had a system in place to verify and reimburse funds so you, basically, took that process, exposed it and made it digital – more self-service?
Chesire: Unfortunately, when you get into these larger organizations, you learn it's not that straightforward. There's usually a series of systems that track your current accounts, as they call it in the UK – it’s your checking account. There's also a series of systems that do transfers, and another series of systems that interact with other banks.
When you call in, the agent first verifies who you are and once they complete that process, they ask your account status, etc. While they’re doing that, they’re “swivel chairing” or “alt-tabbing” over into the transfer system. The minute they see it's to a third-party bank that complicates things.
Just to perform what you think is fairly intuitive task they often have to span dozens of different systems. That's a non-trivial task. When they say, ‘I can't help you, call back in a couple of days, we'll have an update,’ that's because they're sending it to the transfers team, or the interbank team, or something to that effect. And, of course, that leaves you the customer pretty frustrated. So what we’re trying to do is remove some of the friction in that process.
NJ: So you’re bridging silos, basically? If so, what does that process look like when you guys come in?
Chesire: That’s literally one of the terms we use. What we find most effective is working with the business, and usually their peers in the organization who may be a customer service leader, and we ask who else they need to be successful. We’ll then literally map those key business processes and usually it spans many teams and departments.
That’s why we use the term front, middle and back office, because the front office may be the branch or customer service teams. But there are many other teams. And when defining a process, I joke that if you can draw it on a napkin, you can build it on our platform through a visual workflow builder. The ultimate effect is you have an agent workspace that either embeds the information in there or launches specific tools.
One of the things we do is acquire lots of technologies, but we turn them native in our platform. So as an agent you hit a button and the RPA [robotic process automation] goes and performs a task in that other system even if it does not have a modern interface. Regardless, we support all the modern interfaces and integrations up to and including an RPA that may need to pretend to the other system like someone is keying in certain things.
NJ: What is an RPA?
Chesire: Robotic process automation. Think of it like a macro back in the days of Word where you would record a series of clicks or actions – click here and perform this lookup process. I love my banking systems, but some of them were not built for the modern era. So in any industry we obviate the need to necessarily have a modern system – maybe they've grown through acquisition and across various geographies, so you'll get a disparity in systems.
NJ: OK, so where does AI fit in?
Chesire: So as I described, a core part of what we do is automation. We think automation is key to getting customers what they want, but it also relieves the burden on employees from doing repetitive work. We've also been on an AI journey for quite a number of years.
(Editor’s Note: The following graphic is from ServiceNow’s Vancouver press briefing. It shows the acquisitions ServiceNow has made to bring ML/AI into its platform. Vancouver is the name of the software release.)
Chesire: We acknowledge the [use of] new technology and [if] we acquire it, we always take the time to re-platform it so it appears native on our platform. We see [opportunities] for both predictive and generative AI.
One of the non-generative AI produces I'm most excited about is Document Intelligence, which we acquired. It reads documents – an image that you upload or a PDF – and automatically extracts the information, puts it into a form and can initiate a workflow. The AI in it needs a fairly short amount of time to train on a type of document – a receipt, invoice or a deposit slip. That [process] used to be [done by] a human who would have read an image and rekeyed it.
(Editor’s Note: Document Intelligence is based on technology acquired from Element AI.)
We look for those repetitive processes that could be error prone and where the human is not really adding a lot of value. [Automation and AI] can dramatically alter [those types of] processes. You still let the humans take care of the hardest challenges – maybe when it's a large [dollar] amount, a complex transaction, or a high value client. That's where they focus, not on rekeying information.
[When you start] defining a business process flow for performing something you [can] extend it to include things that are already fairly intuitive and built in – [like to check imaging] – so you train the system on images and it starts to do it. And then you can say for this [next] branch in the workflow, I need to go to another system. [Maybe that’s done] with robotic process automation [similar to] what I described earlier. By being native in the platform, we can more quickly implement and get to benefits. In the case of Lloyds Bank, it was only 12 weeks, which – believe it or not – in the business realm is record time to seeing a first productive use and benefits.
NJ: Let’s say an organization doesn’t have any kind of this AI or automation technology in place – how do they benchmark those benefits?
Chesire: AI is simply one method we use to drive outcomes. A key part that we work with customers on is on benchmarking the outcomes they're driving currently for their customer service processes. Then we look at some snapshots in time as they go forward to see the improvement. Lloyds Bank was fairly esoteric – I don't remember the last time I had a bank issue, for example.
But, we did see a dramatic improvement with 7-11 when we worked with them. A few years ago they rolled out a mobile app in which customers could use reward points to purchase drinks as one example. If the customer ran into an issue, they would be interacting with a ServiceNow system. We helped them with categorization and automation and that helped eliminate the root cause of some of the [reward point redemption] issues customers were experiencing – they had a 93% reduction in case volume.
Some of the things we challenge customers on is case volume – it may not necessarily be a good metric – since those cases are where your customers have poor experiences. The challenge is in identifying the issues and figuring out how to eliminate them. 7-11 looked at their mobile app, then at their rewards program, so every case was an opportunity to improve the program and their customers’ experience.
NJ: All of what you’re describing heavily relies on data, having good data and keeping it updated and clean. If I don’t have any type of ServiceNow system or AI/automation in place currently, do I have to change the way the way in which I've stored my data or categorized it to take advantage of the new capabilities?
Chesire: That's simply part of the implementation process. We highly encourage people not to change anything for that current state, but to simply get to a means of at least counting the activities they perform – counting how many emails they get and how many phone calls they get, though some others like chats or SMS may be a little harder,
To help, we sometimes do what we call a customer experience workshop with customers. Basically we just help them audit where they are. As they implement our platform, the beauty for us is we turn everything into a discrete task in the workflow and that can be measured. From that point on, we can fairly easily show improvements.
But it's not a one-time improvement, as I've indicated. As they run on the platform, we encourage them to look at it in terms of continual improvement. So, you first started taking phone calls? Okay, which ones can you automate? Which ones can you embed into the app so that someone can automatically request a missing points credit, for example. And then they see dramatic improvements over time.
NJ: You already mentioned it in the banking example, but AI is good at doing rote tasks and removing humans from performing some tasks can reduce error. Are these areas in which you see most AI tools being deployed? Are there different ways of looking at AI deployments?
Chesire: We think of it in three ways. The first is what you alluded to, which is just making the process automated in the background.
The second is our chatbot – a virtual agent – that's been out for a while. [That agent makes] it tremendously easy for a customer to give an unstructured request conversationally, especially through these digital messaging methods and SMS or WhatsApp if you're outside the U.S. which then turns into a structured request for us.
(Editor’s Note: This post summarizes the difference between unstructured and structured data. And see this No Jitter series on intents and entities.)
The third is supporting the agents who are interacting with the system – they have to do something at some point during the customer interaction. For example, assume a chatbot cannot resolve an issue not because it wasn’t designed to or because it didn't understand it, but because it’s an urgent issue which really needs an agent. We're launching a product that [incorporates] generative AI and will help the agent ramp up fairly quickly when they get that interaction [passed to them].
That [forthcoming product] will summarize that entire conversation [with the chatbot] into a chat summary and gen AI will bring that into a few lines so that the agent understands exactly what happened. For more long running, complicated cases we’ll also be doing case summarization when it needs to be handed off to another agent – let's say a shift change happens and the next team is coming over.
So those are the first incarnations of things we're doing with generative AI summarization – and we’re also doing knowledge article creation. Everybody knows they need quality knowledge or articles, but creating those out of a series of cases into a concise article is something else we're excited about.
NJ: Data security, privacy, PII, etc., are all hugely important with AI and generative AI in particular. ServiceNow has obviously been working with customer data for years, so what is ServiceNow doing to make sure that customer data remains secure, is not used to train somebody else's model or to benefit other companies?
Chesire: We have had our own data center approach for years. And of course, we have strict PII and other data segregation. In our regulated government entities, for example, there's quite a bit of information that already is encrypted at rest and at the column level in the databases in our data centers that even we cannot access.
More recently, we've been working with [Microsoft] Azure for highly regulated industries – the public sector, for example, in the U.S. with FedRAMP. We are very familiar with data security and data privacy requirements – they’re a part of what we do.
(Editor’s Note: ServiceNow’s overarching privacy and data policies can be found here. More information about ServiceNow’s data processing and protection around Now Assist – their generative AI product line – can be found here. ServiceNow said that overall, the workflows and core data structures powering IT Service Management (ITSM), as one example, are anonymized, and customer data and security policies are always considered when ServiceNow provides instructions to a large language model (LLM). The LLM only receives information that is extremely specific to their instance and relevant to the given context.)
We also still see a need for training models and that’s what led to our Nvidia partnership – the ability to build models tailored to customers’ own domains. We’re building [and have built] security, privacy, regulatory requirements at the government- and regulated industries-level into all of that.
NJ: Another question on large language models (LLMs) – you mentioned the Nvidia deal which involves creating customer-specific models. Can you go into some detail there?
Chesire: Everyone knows large language models are trained with [massive amounts of] Internet data and trillions of vectors. Then you get to our platform level with, for example, text to workflow and text to code.
[After that] it gets down to the customer level. We do ask customers to help us train the models, but that is an opt in model. We're also experimenting with smaller models that are tailored to a particular organization's requirements, like, for example, the largest healthcare payer organization in the U.S. Their needs are fairly distinct to them, so we’re working with them to perhaps build a model that's purely for them.
I think another colleague of mine said: We're not in the LLM arms race, but we are fiercely focused on business outcomes for our customers.
Do You Want to Know More?
Check out the other conversations in this series:
Cisco's Lorrissa Horton, who talked about the company's approach to data security in AI.
Google Cloud’s Behshad Behzadi, who spoke about the use of generative AI (Gen AI) at Google in its Contact Center AI offering and, more broadly, across enterprises.
Amazon Connect’s Pasquale DeMaio, who discussed Connect’s history of AI usage and how it’s used in their contact center offering.
Calabrio’s Joel Martins, who talked about current AI implementations in auto-scoring and scheduling and provided a glimpse of how generative AI can help contact center managers, and
IntelePeer’s Frank Fawzi who discussed how current AI implementations can be used to triage customer interactions before they reach the contact center and how generative AI can further improve that process.
Genesys’ Brett Weiglwho talked about how current AI implementations and generative AI can be used to improve the customer and agent experience.
Cognigy’s Philipp Heltewig on the bearing generative AI has for Cognigy’s AI platform, and how his company’s approaching the incorporation and use of that new tech.