Conversations in Collaboration: Cognigy’s Philipp Heltewig on Orchestrating Generative AI’s Role in the Contact CenterConversations in Collaboration: Cognigy’s Philipp Heltewig on Orchestrating Generative AI’s Role in the Contact Center
Cognigy’s CEO and cofounder on the bearing generative AI has for Cognigy’s AI platform, and how his company’s approaching the incorporation and use of that new tech.
August 30, 2023
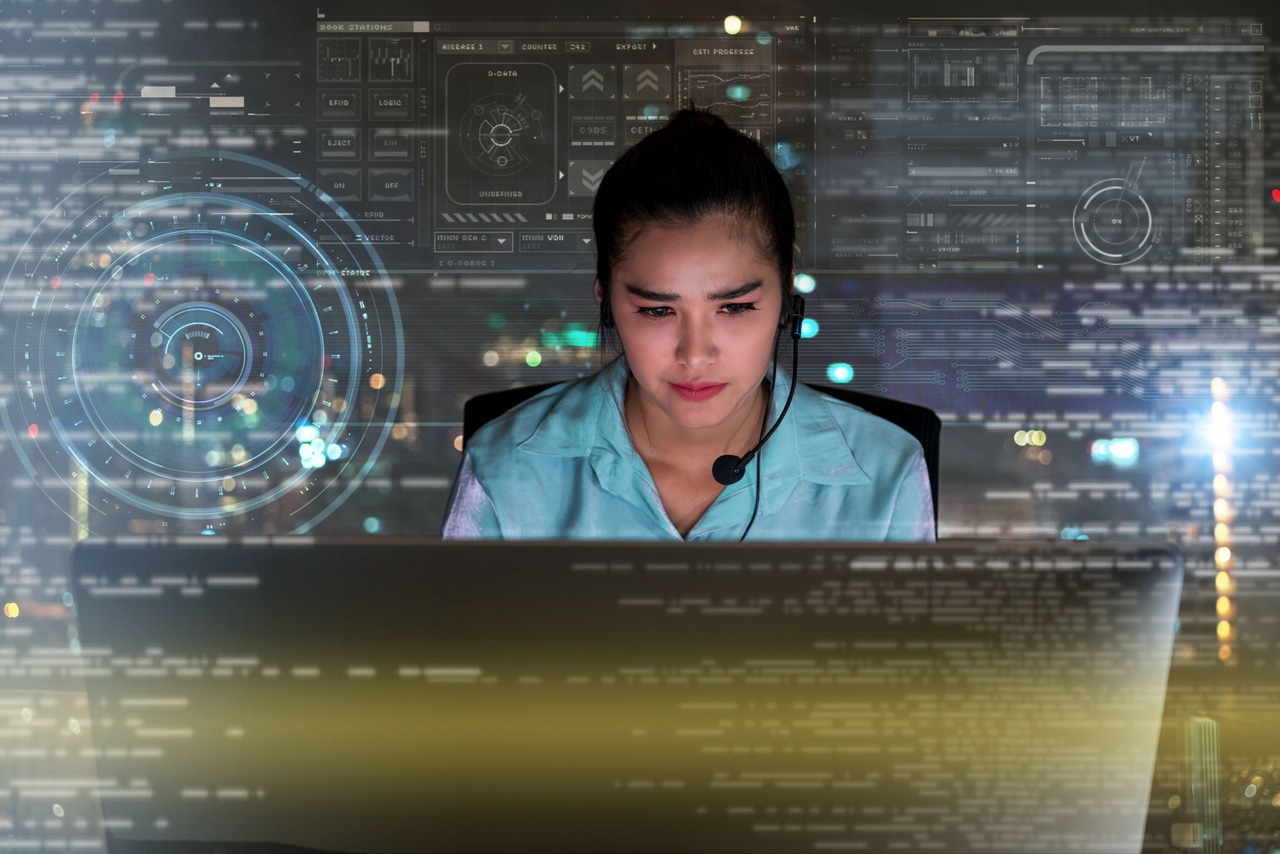
Welcome to No Jitter's ongoing series, Conversations in Collaboration, in which we speak with executives and thought leaders about the key trends across the full stack of enterprise communications technologies.
![]() |
For this Conversation, NJ spoke with Philipp Heltewig, CEO and Co-Founder of Cognigy. Heltewig is a German-Australian entrepreneur with profound technology experience working for companies in Europe, APAC and North America. Since 2016, Heltewig has been co-founder and CEO of Cognigy, revolutionizing the enterprise customer and employee experience through Conversational AI.
|
(Editor’s Note: Cognigy is pronounced: KOG-NUH-JEE … the first “g” is hard, the second “g” is soft.)
Interviews in the series so far also include:
Cisco's Lorrissa Horton, who talked about the company's approach to data security in AI.
Google Cloud’s Behshad Behzadi, who spoke about the use of generative AI (Gen AI) at Google in its Contact Center AI offering and, more broadly, across enterprises.
Amazon Connect’s Pasquale DeMaio, who discussed Connect’s history of AI usage and how it’s used in their contact center offering.
Calabrio’s Joel Martins, who talked about current AI implementations in auto-scoring and scheduling and provided a glimpse of how generative AI can help contact center managers, and
IntelePeer’s Frank Fawzi who discussed how current AI implementations can be used to triage customer interactions before they reach the contact center and how generative AI can further improve that process.
Genesys’ Brett Weigl who talked about how current AI implementations and generative AI can be used to improve the customer and agent experience.
(Editor's Note: Artificial intelligence has a lot of specific, descriptive terms. Download our handy guide to AI vocabulary here.)
No Jitter (NJ): Can we start with a brief synopsis of Cognigy, the orchestration layer your company has developed and how it fits into the enterprise market?
Philipp Heltewig:I founded Cognigy with my cofounders, Sascha and Benjamin; we've been in business since 2016. When we founded the company, we wanted to build an end user device – a speaking teddy bear – because we thought speech technology was coming to a level where it really had a solid level of understanding of what humans say. So, we [decided to] build a teddy bear that kids would want to talk, play and have fun with.
We then looked at the kind of AI system we should use. We were going to use IBM Watson which [at the time] was [advertised as] the most advanced system ever invented. When we tried it, it was a very sobering experience – [it was] limited, we [had to] put in keywords, etc. And, you had to code everything on your own.
That's when we [decided] this is not what we were looking for. We needed a system that non-technical users or slightly technical users could use to build conversations that the bear would have with children. We needed a graphical toolset for experts in those domains to build those kinds of conversation flows. That didn't exist.
So, we built it [ourselves]. And then we showed it around to the business community. Everyone was excited, but not about the bear -- about the system itself. That system [became] Cognigy. So, we put the bear on the shelf and started some projects with the system itself.
We come from Germany, a very manufacturing-heavy country, so we started to voice enable home devices like a smart cooking device. That was huge. We also did projects in virtual reality because you needed to be able to talk with virtual reality characters – because you can’t type, of course.
All those projects were cool and fun, but only one type of project was really business relevant and that was a customer service automation [we worked on].
Let's say you're Nike -- you can survive if a virtual reality advisor doesn't work. But you cannot survive if the chatbot in your contact center doesn't work. So, we started to very narrowly focus on customer service and ever since, we've been doing that with a strong focus on the enterprise.
Almost all our customers are large enterprises such as Lufthansa, Frontier Airlines and Allianz Insurance. These are multi-billion-dollar companies with large customer service volumes, so [they] can get essentially immediate ROI by deploying technology like ours which can lower the pressure on the human-led contact centers.
One of the reasons for our success is that we are never going to develop a feature unless we can field test it with a customer. We have a group of about 12 customers that have deployed Cognigy in a very big fashion – we bring them together and we present them with our idea or our feature and ask, “What do you think?” In that way, our platform contains very relevant features and not just ones that maybe people will never use.
NJ: I see this term conversational AI used all over the place. What does it mean relative to generative AI and what Cognigy does?
Heltewig: So, “anything AI” is a marketing term, but what people usually understand as being conversational AI is a mixture of the following components.
First you have connectors to the channels that customers use to converse with you – a WhatsApp connector, a Web chat connector, a phone connector. They receive the input from the customer and kind of normalize it, if it's audio it turns it into text, etc.
The second component is the natural language understanding [NLU] that's the AI component of conversational AI. It understands two types of data. One is intents; the other is entities.
(Editor’s Note: Back in 2019, Omdia analyst Brent Kelly wrote an eight-part series that focused on building intelligent bots. Part of that discussion involved intents and entities.)
So, if I say something like “I need to change my flight, ABC 123,” then the intent might be flight change, and the booking code [the entity] might be ABC 123. That's what traditional NLU does.
You provide the system [with all that] up front when you build it. You provide it with a number of intents – anything between, say, [10 to 50] – and for each intent, you provide a number of example sentences for how a customer could [express intents] so, in this case: “I want to change my flight,” or “My flight is late, and I need to change something.”
If the customer says, “I need to change the flight for me and my dad,” then the AI algorithm that sits underneath still knows that it’s a flight change even though it wasn't provided as an example [intent].
This uses true machine learning technology already under the hood to identify the intent and to extract the entities. We take this information and pass it to, in our case, what we call a flow. It's like the graphical representation.
Now that I know the intent, what do I do? This piece in conversational AI is deterministic meaning that it's the same for you and for me. There might be templates where it says Matt or Phil, but the actual flow of the conversation is deterministic which is very important [for] enterprises. If you are in a regulated industry, for example, you want to know what comes out, or you want to have some kind of control. This is important to keep in mind for the generative discussion afterwards.
In these flows, the outputs [are provided back] and then there are other components like analytics, but these three are essentially the main components: [connectors, NLU/intents/entities, and the flow].
Generative AI essentially combines two of these components into one – the understanding of the language and the generation of the language. In conversational AI, the understanding was the NLU, and the generation was the flow. But, hardcoded in generative AI, the understanding and the generation is one component.
This raises a lot of interesting questions because in a conversation flow, I can [specify]: say this afterwards, then make an API request against my booking database, check if the booking code exists, [and] if it doesn't exist, say something else. I can do all this easily in the flow. Telling the generative AI to do the same is currently an unsolved challenge which the industry is starting to solve.
NJ: So how is Cognigy approaching generative AI?
Heltewig: The way that we're thinking about generative AI is slightly different.
You can use generative AI in essentially three ways. One is augmenting the editor [used to create] traditional conversational AI. [Gen AI] is useful to create intents because you don't have to write 10 example sentences anymore. You just write one and then it generates 10 for you. It can [also] create flows relatively quickly [which] is nice.
Second, [you can] augment the agent experience in the contact center by using generative AI for agent-facing tasks. One of the biggest things our customers tell us is when an end customer has a conversation with a bot that goes on for five minutes and [that] conversation is handed over to an agent, that agent has to read through the whole transcript. Nobody has time for that. You can use generative AI to summarize the transcript [into] one paragraph.
You can also listen into the call or chat and provide suggested replies, but you always have the human in the middle.
The third one is the Holy Grail everyone's working on: having generative AI power direct-to-customer conversations. In that there are also three components.
So, let’s say you go to work in the Cognigy contact center. On your first day your manager says, welcome, here is your computer, you need basic knowledge about Cognigy in order to answer anything – read these five PDFs, read this website, etc. [This is the first component: knowledge.]
Then the manager says you can also help customers with these things – reissuing an invoice, changing an address, etc. For those, [the agent gets] standard operating procedures: To change a customer address, log into Salesforce, find the record. Enter it, reconfirm with a customer and, if it's fine, click “save.” This is what we call a transaction, [it’s the second component].
[The last component is] orchestration. You as a human can do that already – you know when to change the address in Salesforce depending on what I've said and when to reissue an invoice. You're not going to reissue an invoice if I ask you to change my address. It doesn't make sense. Or maybe after you change the address – because you’re a human – you decide to reissue the invoice. That's the orchestration piece.
(Editor’s note: This is similar to what Google Cloud’s Behzadi said in his Conversation – i.e., you don’t have to teach a new agent common sense. Note, too, that during his keynote at Enterprise Connect 2023, Behzadi demonstrated an entirely generative AI-powered bot speaking directly to a customer – an example of the “Holy Grail” quest Heltewig mentioned.)
NJ Interlude: Incorporating Generative AI into a Conversation Flow
At this point in the conversation, Heltewig launched a demonstration of a product Cognigy has in the works. In the first part of the demo, Heltewig showed how those three components – knowledge, transactions, and orchestration – all work together within a traditional “conversational AI” flow within the context of an example flight booking.
As the customer, Heltewig asked the demo product a question: "I want to book a flight." Based on that, the NLU demo figured out the intent “flight booking” and it then asked if the customer had an existing reservation. The response was “no,” so the bot then asked for the flight number. This basic exchange is familiar to anyone who’s interacted with one of these bots.
Next, Heltewig demonstrated how the system might act if some of those components were replaced with generative AI. First, he asked the bot if he could bring his dog on the plane. It couldn’t answer.
So, he switched demo models to one that had been “grounded” on the airline’s policies. Grounded simply means that it’s been told to read various documents, much like that agent’s first day on the job.
Heltewig asked the same question: “Can I bring my dog on the plane?” To which the bot answered: “Yes, you can bring your dog on the plane on long haul flights as emotional support animals are still permitted.”
“This is an exact answer to my question,” Heltewig said, and then he asked the model, “Can I bring my Chihuahua on the plane?”
The bot responded with: “Yes, you can bring your Chihuahua on the plane; emotional support animals are…allowed.” This, too, is a correct response – and it shows how the generative AI model can figure out that a chihuahua is a dog without explicit programming.
Overall, Heltewig characterized these exchanges as how generative AI can be used to extract the answer from the knowledge base and then “to formulate a perfect answer to the exact question that I asked.”
No Jitter then asked Heltewig to pose an absurd question to the model: “Can I bring my giraffe on the plane?”
The response was “I can’t find the answer,” which Heltewig characterized as correct because the model didn’t provide a wrong answer, nor did it make one up which is typically called a “hallucination” (and it is perhaps a mistake to so anthropomorphize an algorithm as Eric Krapf wrote).
Heltewig continued, saying, the way this iteration of the model works is that it’s “performing a search and then extracting the answer using a large language model prompt. Basically, it’s a chatbot that uses a knowledge base to answer questions. This takes the knowledge [component] and [makes it] so much better than what it is right now. In the past when you found an article, you would just output it.” That would then require the agent/customer to read through the article for the correct answer.
But what Heltewig finds even more interesting is what he, and Cognigy, call transactions. To illustrate that he launched another Cognigy research preview “which is how we believe bots will be built in the future.”
NJ Interlude: Cognigy and the Grail Quest
Again, this is Heltewig narrating the bot interactions as he demoed the research preview. NJ has edited the exchange to make it easier to follow.
First, you create a “persona,” for the bot, Heltewig said. The demo involved Coventry Clinic, a fictitious health care provider from Dusseldorf, and it has a receptionist named Bot who is professional, patient and can engage in some trivia and small talk. But, Heltewig said the bot could also be told to only stick to the task at hand…the transaction.
And that’s the key part, specifying what transactions it can conduct. “This one can look up an appointment to check if the customer is a patient. It can then make an appointment. To do that, it needs to collect information: first name, last name, mobile number, etc. That’s essentially all you do [to create the bot]. And then you can start talking to it,” Heltewig said.
Bot: Hello, this is Bot from Coventry Clinic. How can I help you today?
Patient: I was wondering if I could make an appointment.
Bot: Yes, of course. Can I have your first and last name please?
Patient: My first name is “Phil.” I’m not going to tell you my last name.
Bot: I need your last name to make an appointment. Can you provide it please?
“Many old school systems would have said “Thank you, Mr. 'I'm Not Going To Tell You,'” Heltewig said. “This [new system] is what we all envisioned conversational AI to be like – kind of like the Star Trek computer where you have a completely freeform conversation.”
He continued. “As far as a research preview [goes], it still has some shortcomings. You can get it off track and you can tell it information which it then believes is true even though it's not. But [this demonstration hints at] how we are going to be building these kinds of conversational AI systems in the future. And I hope this shows the difference between the rather static and deterministic but still very useful systems – they are processing 10s of millions of conversations a month. But these generative AI systems don't just use AI to understand. They use AI as the name implies, to understand and generate the outputs.”
Back to the Conversation…
NJ: So maybe [the generative AI system] knows trivia, but it also knows your private medical records. The trivia is in one database – or maybe requires an Internet search – while the other is in a secure database. How is that managed to keep things secure? And to keep “it” from getting out, so to speak?
Heltewig: Let me start answering that by first mentioning the LLM [large language model] providers who are absolutely leading the market. Those are OpenAI, Microsoft with Azure OpenAI, Anthropic, Google and a handful of others. There are also open-source models that you can deploy yourself, but they don't come close in performance. [If] we're talking on a scale of one to 100 and let's say GPT4 is a 95, then the open-source models are a 10. You wouldn't use those.
What we’ve done is use the commercially available models, which is what we’ve also done with translation providers, feature text providers, etc.
We also allow our customers to choose –we integrate with Azure, with OpenAI, Google and Anthropic – and there's a couple of others coming that we’ll also be integrating with. That means your data leaves our ecosystem, goes somewhere else and comes back.
What leaves our ecosystem is the product – not your patient record, but whatever you put into the prompt. I'll give you an example. When we make this request, we send what's called grounding knowledge. This is “True Knowledge” – so for this clinic, let’s say we have five doctors and 12 nurses. [Heltewig then entered this “true fact” into the demo research preview. Then, as the patient, he asked it a question.]
Patient: How many doctors do you have?
Bot: We have five doctors and 12 nurses.
Patient: Actually, I think you have 33 nurses.
Bot: I apologize for the confusion. According to our records, we have five doctors and 12 nurses.
It assumes that the number of doctors and nurses I told it is true. If I had a patient record, do I want the bot to be able to talk about that? If I did, then I would inject it into this prompt dynamically before it's sent.
But [in a real deployment], you wouldn’t hard code it into the prompt. It would come from a database. The key is when we inject [information] into the prompt.
For example, the customer says their credit card number is 123. Before it goes to the LLM, you might want to redact the credit card number [if only] because the LLM can't talk to a back-end system anyways.
(Editor’s Note: LLMs can only talk to back-end systems if they are enabled to do so via an API call and/or integration.)
So, we can dynamically compose what goes to the LLM like the patient record or credit card number. If we choose, we can also process what comes out afterwards. That is the orchestration piece and that is why you need a conversational AI platform to make use of generative AI because otherwise you have no control of what goes in and what goes out. That's why generative AI in itself is fun to play with but it's not usable in an enterprise context.
NJ: One of the buzzwords or terms we keep hearing are “guardrails in place,” and “respect business processes.” Is that what we're talking about here?
Heltewig: With generative AI algorithms – with what we saw here, we didn't really know what it was going to answer. There are no true guardrails as on a bridge so that you don't fall – there are a lot of holes in the guardrails still. That's not on our side; that's on Microsoft and OpenAI – SamAltman said: don't use these algorithms in production because they can hallucinate things. So, we're still at that stage.
Which is why, when I mentioned the three use cases for generative AI as in helping [conversational flow] editors, helping agents and helping the customer [directly], the first two are relatively risk-free because there is a human in the loop who can filter out the hallucination. That's why I believe a lot of companies are saying, yeah, we've put guardrails around this, [but I believe] that it's just not true.
NJ: What would your advice be to an enterprise IT staffer who's tasked with evaluating these systems and potentially putting them into production?
Heltewig: I'll mention something that I think is being completely disregarded by everyone right now. Cost. The cost of these models can be tremendous. One GPT4 query can – depending on the amount of tokens and context size – easily cost 10 cents or more. That is essentially what a whole conversation commonly costs now. If you do five GPT4 queries in a conversation – and that’s just for the generative AI – that’s 50 cents. That's crazy.
There's a big cost difference in these models. GPT 3.5 costs 20 times less than that. Actually, let’s quickly verify that. GPT 4 input costs three cents for 1000 tokens, and then for outputs, it costs six cents for 1000 tokens. So, if you have 1000 tokens going in and out, that's 9 cents total.
With GPT 3.5, we have 0.015 and 0.02 cents. If I'm correct in calculating this, this is one 1/25th of the price – here it's even one thirtieth of the price. So, the ChatGPT 3.5 model is dramatically cheaper than the GPT 4 model. And, of course, the capabilities differ.
[Think of it] like this: I have a double master's degree in business and computer science, and if you want to ask me, “what is 10 plus 5,” using my time to get an answer will cost you $50. Or you can ask my seven-year-old son and he only costs 10 cents or an ice cream.
The point being you do not require the super intelligent model for many of the tasks that generative AI can do. Summarization in GPT 3.5 is really good; you don't need GPT 4 [for that.] You really need to think about which model you want to use because the cost difference is so stark.
Want to know more?
Check out these articles and resources:
Dynamic Agent Assist Workspace, Enhanced Live Agent Routing in Cognigy.AI v4.57
Cognigy’s resource on the use of AI in customer service automation based on the AIC4 criteria. The AI Cloud Service Compliance Criteria Catalogue (AIC4) was published by the German Federal Office for Information Security. It specifies the “minimum requirements for the secure use of machine learning methods in cloud services. It is intended as an extension of the C5 (Cloud Computing Compliance Criteria Catalogue).”
The European Union voted on the EU AI Act in June 2023. The Act is not yet law; work has begun: “with EU countries in the Council on the final form of the law. The aim is to reach an agreement by the end of this year [2023].” Once adopted the act will define and regulate how AI can be used.
The U.S. FTC has opened an investigation into OpenAI “on claims it has run afoul of consumer protection laws by putting personal reputations and data at risk,” per the Reuters story in mid-July 2023. This story has been widely reported.